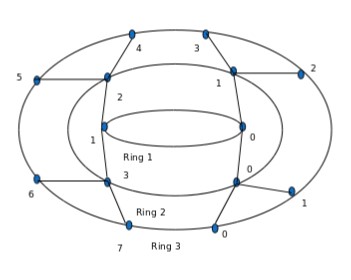
Field programmable gate array (FPGA) is become one of the best way in looking the functionality of a integrated circuit. We can download any logic to an FPGA and test the logic quite easily and then if the design is correct then we can go in for an ASIC design if required. Also if the logic is going to change very frequent then the logic can be downloaded to a FPGA and used a chip.
Network on chip is a new dimension in VLSI design wherein we use a network for transfer of information rather than a bus structure which would be slow in working as the logic of implementation goes high. Many topologies like mesh, torus etc. were introduced area of network on chip in the beginning. These topologies became slow when the logic of the system grow. For this a new topology called RiCoBiT (Ring Connected Binary Tree) was introduced in this area. This topology is better in terms of the hop count by keeping the area the same as mesh or torus.
The project we are doing, will give a new dimension for FPGA based design. Here we are going to use the concept of FPGA with network on chip. We are designing a new reprogrammable device like a FPGA using RiCoBiT topology.
Demo Video
Project Proposal
1. High-level project introduction and performance expectation
Field programmable gate array (FPGA) is become one of the best way in looking the functionality of a integrated circuit. We can download any logic to an FPGA and test the logic quite easily and then if the design is correct then we can go in for an ASIC design if required. Also if the logic is going to change very frequent then the logic can be downloaded to a FPGA and used a chip.
Network on chip is a new dimension in VLSI design wherein we use a network for transfer of information rather than a bus structure which would be slow in working as the logic of implementation goes high. Many topologies like mesh, torus etc. were introduced area of network on chip in the beginning. These topologies became slow when the logic of the system grow. For this a new topology called RiCoBiT (Ring Connected Binary Tree) was introduced in this area. This topology is better in terms of the hop count by keeping the area the same as mesh or torus.
The project we are doing, will give a new dimension for FPGA based design. Here we are going to use the concept of FPGA with network on chip as the back bone. We are designing a new reprogrammable device like a FPGA using RiCoBiT topology.
The purpose of this design is to have high performance algorithms to be implemented on ASIC / FPGA design. We can code a high performance algorithm on Verilog and then download the same and work with this FPGA. The application areas can be high speed DSP, defence application, space application etc. The users of this FPGA would be defence scientist, space scientist, high end solution design architect etc.
From the view of Intel, it can use this for their design for the manufacturing of their next generation FPGA. This generation of FPGA’s would be faster and will be more efficient in the working. For this the FPGA and the tool for programming this chip should be developed.
2. Block Diagram
Fig 1.1 RiCoBiT Topology With Three Rings
The proposed RiCoBiT topology resembles the spider’s cob web as shown in figure (Fig 1.1) The topology consists of growing interconnected concentric rings. The rings are numbered from 1 to K where K is the number of rings in the configuration. The number of nodes in ring L is 2L numbered from 0 to 2L - 1. Therefore, the number of nodes Nr in the configuration with K rings is
The node n in ring L is connected to its neighbouring nodes 2n and 2n +1 in ring L + 1. The number of wire segments to connect the nodes in ring L to those in ring L + 1 are therefore 2L+1. Similarly the nodes within each ring are also interconnected by wire segments. The number of such wire segments in ring L is 2L.The proposed topology has several advantageous properties. The topology is symmetric and regular in nature. It is structured, modular and scalable limited in size by the fabrication technology. The scalability property is well complemented with high performance with marginal increase in chip area.
3. Expected sustainability results, projected resource savings
The design is still in the beginning stage, so no performance parameters is possible now. The topology RiCoBiT is tested with the counter parts – mesh and torus and RiCoBiT produces better results in terms of performance (Max Hop & Average Hop) keeping the area parameters (Wirelength, No Of Wire Segments) almost the same. So building a FPGA with network on chip as a background will definitely be advantageous.
The product is a research work carried out in REVA University, Bangalore, India. If Intel is interested, we are ready to pass on the patent and copyright filed in India to Intel for manufacturing.
4. Design Introduction
The design is a new type of reprogrammable device like a FPGA wherein the users can reprogram the device any number of time to realise their logic. The application with this device can be where use of high speed processing like military, defence, scientific applications. The targeted users will be application engineers and system development people looking for high performance in their application.
This work does not require a FPGA for testing. We are designing a new device which will be work like a FPGA with network on chip as the back bone. For this we are using RiCoBiT, which is already proven to be better than mesh, torus and other topologies.
5. Functional description and implementation
The design is related to a new reprogrammable device like a FPGA but this device will be using a network on chip topology for routing. Here the design of the logic to be implemented would be in such a way that it would exploit the parallelism in the code.
We proposed to make the device with RiCoBiT topology which is proved to be better in terms of the hop count than other topologies like mesh, torus etc. The RiCoBiT topology is better in terms of the area parameter also. We plan developing a device with 128, 256, 512 or 1024 nodes.
6. Performance metrics, performance to expectation
The overall speed of the device would be the major criteria in the project. It is already proved that network on chip would be better in terms of performance than common bus architecture. Here we are replacing the design using network on chip based topology i.e. RiCoBiT which is already proved a better option in terms of maximum hop and average hop (https://ieeexplore.ieee.org/document/7411165)
With RiCoBiT, we plan to build the reprogrammable device. Regarding the area consideration, it is proved that the area is almost the same as taken by mesh and torus. Even though there would be an increase of about 4% in area it would not be a big point as we are getting better performance.
Also there is no comparison made with Intel FPGA’s as this concept is a new invention.
7. Sustainability results, resource savings achieved
We plan creating a C / Java simulator implementation of the device. Later if the simulator gives better performance then Verilog code of the design would be done to verify the functionality of the device. We can include all the features required in the simulator so as to know the overall working of the system
8. Conclusion
The RiCoBiT topology is explained and a non-adaptive and adaptive algorithm for RiCoBiT topology is developed and their implementations with results are discussed. The internal structure of the reconfigurable device which is proposed to implement using this topology is introduced here.
0 Comments
Please login to post a comment.