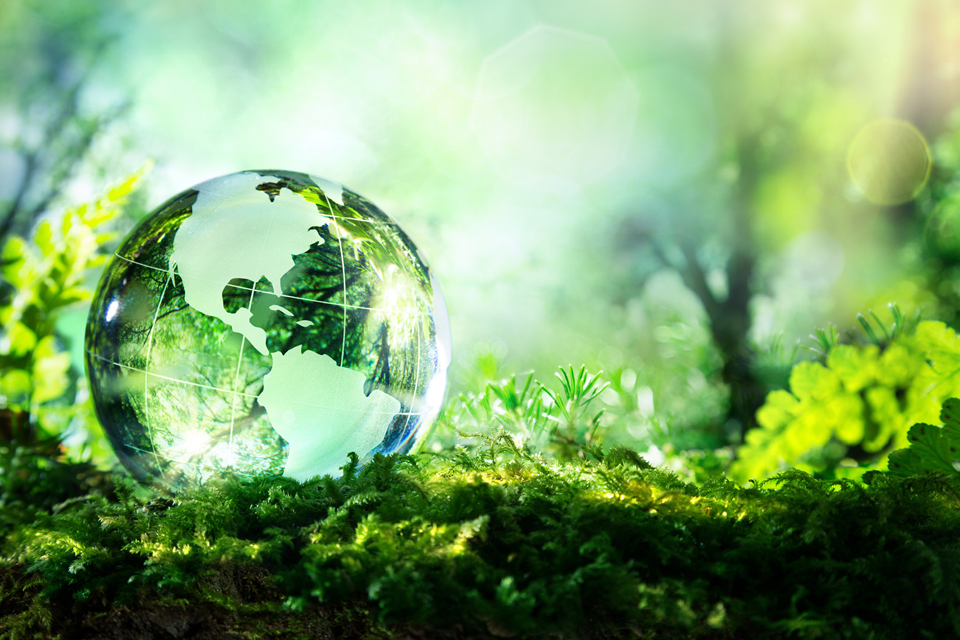
Over-Watering and Under-Watering are the two concern-able scenarios actively addressing the water conservation which is one of the major global challenges. There are trained gardeners who can water the plants regularly and maintain the gardens and other landscape plants effectively. But how much we control the water wastage from human hands while watering plants, there is a considerable error happening always, and we can control it by autonomous systems. Project "Green-Globe" is our idea to create some momentum in addressing this issue. We're focusing to develop an authentic system with using which we can utilize the natural resources ( i.e., Water) properly to grow the plants effective and efficiently. So, the idea is to develop an autonomous irrigation system to water plants with which we can automatically water the plants by understands and calculating the primary parameters like plant genetic information, moisture in the soil, humidity in atmosphere and secondary parameters like temperature and electrical conductivity of soils. The realistic approach of our project comes in a long run, but it will create a benchmark for the initiation of connecting the edge technology of INDUSTRY 4.0 with global challenges of today. Project Green-Globe delivers the essential requirement i.e., growing the plants effectively and efficiently by regulating the Over-Watering and Under-Watering. The outcome also addresses to saving the water and conscious usage of the water while watering plants at houses and other areas.
The developed system is able to regulate the water wastage, and is able develop ML and AI models from understanding the plant's genetic information and the rate of it's growth, and also using climatic conditions which were collected via Internet APIs and cloud. The developed models helps to perform better autonomous irrigation methods, and could also be helpful at concepts of terraforming other planets.
Demo Video
Project Proposal
1. High-level project introduction and performance expectation
Purpose of design:
Overwatering and underwatering are two concern-able scenarios happening while watering and growing plants. Unfortunately, both cases are having their impressions on some of the global challenges. The plants need their existence to continue to make human beings and other species survive on planet Earth. Overwatering is a very severe case as it is addressing two of the major global challenges i.e., Climate Change & Water Conservation. And Under Watering could fall into an indirect active reason for Climate Change (under global challenges). To maintain sustainability, a conscious and inclusive human effort is needed on increasing the graph of green growth.
The graph of green growth includes the growth of plants that grow in smaller areas like homes, office-landscape areas, and larger areas of crops and forest lands. There is a lot of background work and human effort going on to maintain consistency in making the viable eco-system strong enough to provide resources for the next generations, however, we need more and more optimal solutions to address these challenges. Project Green-Globe is our idea to create some momentum in the addressing aspect of this issue.
General Information about the design:
The overall concern of this project is to accomplish the requirement of growing plants efficiently by regulating the water provided to them. Project Green-Globe is a customized and intelligent watering system for horticulture. With this project design, we are focusing to develop an authentic system with using which we can utilize the natural resource i.e., Water properly to grow the plants effectively and efficiently. So, the idea is to develop an autonomous irrigation system to water landscapes plants with which we can automatically water the plants by understanding the plant's genetic/botanical information and calculating humidity in the atmosphere, ambient temperature, and other weather conditions.
Plant genetic/botanic information is used to collect and understand the plant’s usual behavior of growth and frame the study cases about how much water it takes while growing. The ambient temperature, humidity, pressure, and pressure tendency in the atmosphere are taken for making better predictions of rainfall chances and understanding how much water is supposed to schedule for the delivery to the plant.
Application Scope:
The project outcome expects to minimize water usage by regulating the water control that is provided while watering the plants through an autonomous system.
The scope of this autonomous irrigation system could primarily include:
i. The regulation of water waste and creating an impact on conscious water conservation.
ii. Growing plants effectively by saving resources.
iii. Reducing the dependency on sensors for autonomous systems.
iv. Keeping a record of the and providing supplies and water delivery schedules.
v. Initiating the idea of connecting the INDUSTRY 4.0 technology to conserve natural resources and address global challenges.
Targeted Users:
The system is designed in such a way that any small level pots to large areas of garden/crop level method of growing plants and trees can adapt it, making it feasible to implement.
i. Any scale Nursery.
ii. Gardens at homes and offices.
iii. Landscape areas.
iv. Small to large scale crops of horticulture areas.
v. Scientists with research workplaces on plants.
Why Terasic DE10-Nano development board, and Microsoft Azure Services for this project:
While considering the performance of the device and the capability of logic making, we believe Intel FPGA devices are better for reliable functionality and Microsoft Azure Services for better security concerns. The Terasic DE10-Nano board comes with an Intel Cyclone V SoC FPGA and Hard Processor System (HPS) of ARM Cortex-A9 processor. One more strong reason for picking the Terasic DE10-Nano development kit is that we are attracted by its key benefits of reliability and long-term maintenance which adds an advantage to improve the performance and proper outputs of project Intel SoC FPGAs combine the familiarity of an ARM processor with the flexibility of programmable logic while connecting the device with cloud and perform logic operations. That's what makes us choose Intel FPGA devices with Analog Devices and Microsoft Azure Cloud for this project.
2. Block Diagram
Block Diagram
Algorithm (Flow Chart)
Flow Charts (Logic Blocks Explained)
3. Expected sustainability results, projected resource savings
Considering the performance parameters, our design system is supposed to save water resources while overwatering the plants and provide enough water to the plants that are not getting enough water, basically underwatering. This essentially helps the plants to grow effectively and efficiently. There are trained gardeners to look upon the gardens but how much we try to minimize human errors, there is always a considerable amount of error happens. The project delivers its essential requirement of delivering water to the plants with the autonomous system and the idea is to reduce human interference while watering the plants which can make that error to zero. It is also necessary to keep a record of the performed activities like water schedule timings and plant monitoring units. Even though saving the water resources by regulating overwatering and underwatering is our main focus, the other major goal of doing this project is to create a benchmark for the initiation of connecting edge technology with the solutions to save natural resources and address global challenges. So, the list of performance parameters includes -
EXPECTED SUSTAINABILITY RESULTS:
1) To regulate overwatering and underwatering by creating a conscious usage of water while watering plants.
2) To grow plants effectively with a proper water supply.
3) Keeping a record of the activities performed and collecting data that can be used for further improvements in various models.
4) Reducing the dependency on sensors for autonomous systems, which makes the systems more reliable and cost-efficiency.
3) Initiating the idea of connecting the technology for sustainability by conserving natural resources and addressing global challenges.
PROJECTED RESOURCE-SAVING:
1) Saving water resources by creating an awareness of water conservation.
2) Eliminating the human intervention and manpower while watering the plants.
3) Making autonomous irrigation systems more cost-efficient by providing more better results with less usage of sensors.
4. Design Introduction
Basic Block Diagram for Design Introduction
Design Introduction:
The autonomous system we developed contains 2 control units, 1 cloud unit, 1 internet API block, and an additional plant monitoring unit.
PRIMARY CONTROL UNIT (PCU):
The primary control unit is the main controlling part. The main controlling part of the entire project is processed with the Terasic DE10NANO development board. The board comes with high-speed DDR3 Memory and ethernet networking. The DE10NANO development board consists of two parts.
The FPGA part, and the HARD PROCESSOR SYSTEM (HPS) part. Since the cloud unit and internet play a key role in our project. We have chosen to use the HPS part, which is having an 800Mhz dual-core ARM Cortex – A9 processor with 1GB DDR3 SDRAM (32bit data bus), 1 Ethernet RJ45 port, USB OTG port, USB Micro-AB connector, Micro SD card socket, Accelerometer (I2C interface), UART to USB, USB to Mini-B connector, reset buttons and LTC 2X7 expansion header.
The primary control unit has an additionally attached hardware RFS Wi-Fi module which consists of a 2X20 GPIO Interface, UART to USB, 2X6 TMD header, Temperature Humidity Sensor, and 9-axis sensor with Accelerometer, Magnetometer, and Gyroscope.
SECONDARY CONTROL UNIT (SCU):
The secondary control unit takes the instruction signals from the primary control unit through the cloud unit and performs actuation. The secondary control unit is a Microcontroller with ESP32 Wi-Fi Module. Here, we are using Bolt IoT Wi-Fi Module which comes with 5 digital GPIO pins, 1 analog GPIO pin, 5V, 3V, and Ground Pins.
CLOUD UNIT (CLU):
The cloud unit plays a major role in the entire design architecture of this project. Generally, the cloud means a system with specific resources and storage attached. We are using the cloud for storage. We are storing databases and saving the records of logs of every action performed. The cloud is a two-way communication for both the primary control unit and secondary control unit.
INTERNET API BLOCK:
For predicting rainfall and monitoring weather conditions, we are using some internet APIs. For authentic data, we have chosen Azure Maps and Weather Conditions services.
PLANT MONITORING UNIT (PMU):
The plant monitoring unit is integrated with the secondary unit. The records of water delivery and the soil moisture sensor values from the plant are monitored and saved in the cloud storage point. The plant monitoring unit is a mix of some hardware with cloud and internet.
FPGA virtues in this project design:
To fulfill our project objectives, we need to create robust communication between the main processing unit and control unit so we require a perfect cloud connectivity kit.
Hence to satisfy our requirement we have chosen the Terasic DE10-Nano development kit, featuring an Intel Cyclone V SoC FPGA which is a robust hardware design platform for makers, educators, and IoT system developers. one more strong reason for picking the Terasic DE10-Nano development kit is that we are attracted by its key benefits of reliability and long-term maintenance which adds an advantage to improve the performance and proper outputs of project Intel SoC FPGAs combine the familiarity of an Arm processor with the flexibility of programmable logic. The board includes two 40-pin general-purpose expansion headers and an Arduino (Uno R3) header to support a wide range of sensors, controllers, and displays. The DE10-Nano development board is equipped with high-speed DDR3 memory and Ethernet networking. And it has wide applications in Computers, Broadcast, Consumer, Industrial, Medical, Military, Test and measurement, Wireline, Automotive. We are using the HPS part from the de10 nano kit which is having specs of 800 MHz dual-core ARM Cortex-A9 processor which 1 GB DDR3 SDRAM (32-bit data bus) 1 Gigabit Ethernet PHY with RJ45 connector USB OTG port, USB micro-AB connector Micro SD card socket Accelerometer (I2C interface + interrupt) UART to USB, USB Mini-B connector Warm reset button and cold reset button One user button and one user LED LTC 2x7 expansion header.
Even though the DE10-Nano board comes with ADC, we cannot use the board to work interface it with sensors as we can only implement a limited number of sensors. We focused to use the processing capability of the Terasic DE10-Nano Development board to its full extent and decided to choose it as the primary control unit of the system. Even though we tested the DE10-Nano board by interfacing the sensors and AD Plugin Boards initially, we understand that it was capable to perform the tasks which are required for the primary control unit the processing speed, and the boost performance of the board.
5. Functional description and implementation
Algorithms and Flowcharts:
Description of the functionality:
The primary control unit is the central controlling part and is connected to every other unit mentioned in the block diagram.
The code/program which runs in the primary control unit fetches the APIs for weather conditions and retrieves the ambient temperature value from the RFS module. The data is constantly taken every 10-15 minutes.
The dew point, clouds condition, precipitation summary, real feel temperature, apparent temperature, relative humidity, pressure, wind speed, and direction are taken from the Azure Maps Weather Conditions API to perform rainfall prediction.
The previous and present values of temperature are taken to predict temperature forecasts using linear regression. The collected temperature values were later taken to predict the Z-Score Analysis. The Z-Score Analysis is technically for Anomaly Detection. With the given temperature values, the program constantly updates the Z-Score, and eventually, the bounds are also updated for every data point i.e., temperature value. Based on the bounds, the program identifies if there is a sudden change in the temperature. If there is a sudden drop in the temperature values, the program understands it as an anomaly and then checks for any possible rainfall forecast using machine learning algorithms. If there is a possible rainfall predicted the program will check for the other APIs to fetch the actual rainfall measurements in cm/hr or other metrics.
Later the program flow goes to schedule the timings to deliver the water to the plants accordingly. It is defined in the code initially that the amount of water required for the plants. The date and month are taken to find the current season at that particular location. If it is a torrid season with high temperatures like summer and autumn, the water amount is divided into several times defined as sets, to avoid water evaporation and deliver the plant with the required water.
All these values are pushed into the cloud storage point using the internet. Meanwhile, the secondary control unit checks for the instruction signal sent by the primary control unit, in the database. It fetches the schedule timings and performs actuation at that time. It turns on the motor/water supply according to the runtime calculated.
Implementation:
As mentioned in the “Design Introduction” section, the primary control unit performs all the computation and pushes the data to the cloud. The secondary control unit actually takes the instructions signals (which are the scheduled timing data here) and performs actuation.
To eliminate the dependency on the connectivity of both devices together and to make the systems work with more performance, we made the design in such a way that both the primary control unit and the secondary control unit need not be kept nearby each other. The primary control unit can be kept anywhere in the world and still can perform its job. The secondary control unit can be kept in the field attached to a motor/valve and perform actuation according to the schedule made by the primary control unit.
There are various kinds of garden implementation methods according to the fields and plants they grow. We worked on the implementation aspect to make it flexible and adaptable to any kind of method. We designed the implementation of this system into 4 categories making it suitable for any kind of garden/crop plant.
They are POT LEVEL IMPLEMENTATION, CROP LEVEL IMPLEMENTATION, GARDEN LEVEL IMPLEMENTATION, and MIXED GARDEN LEVEL IMPLEMENTATION.
One is POT LEVEL IMPLEMENTATION. It is made for both indoor and outdoor pots. If the pot is located inside, the relative humidity and temperature values are used for computation and to make the schedule for delivery of the water to the plant that is kept in that pot. If the pot is located outside the temperature and other weather conditions (mentioned above in “Description of the functionality”) values are taken to compute the water delivery schedule. According to the schedule, the secondary control unit will initiate the actuation and deliver the water.
The second is CROP LEVEL IMPLEMENTATION. If it is a crop, there is only one kind of plant. But in more number. So, the botanical data of the plant about how much amount of water is required and the total number of plants is defined in the program initially. The program takes these values every time and calculates the total amount of water to be delivered and delivers that water to the plants using the above-mentioned algorithm.
The third is GARDEN LEVEL IMPLEMENTATION. The same happens with garden-level implementation also. The difference between crop and garden is that there is more than one kind of plant in the garden. All the garden plants are mostly ornamental and almost plants require water of the same amounts. So, we are taking the total number of plants in the garden and the amount of water needed for each plant. Based on the density (which can also be considered as frequency) of each plant, the total amount of water that is needed to supply could be calculated and then the water is delivered by following the flow of the algorithm.
The fourth is MIXED GARDEN LEVEL IMPLEMENTATION. The mixed garden is nothing but, there could be gardens with plants that are not only limited to ornamental plants. Just like we grow fruits/vegetable kinds of plants along with ornamentals at houses. For these kinds of cases, the water supply valves could be separated into two or more. Then the pipeline is separated and laid in more different ways making it feasible to supply water for all kinds of plants efficiently. The pipelines that go along with fruits/vegetable kinds of plants deliver the water to those plants separately and, the pipelines that go along with ornamental plants deliver the water to those plants separately.
6. Performance metrics, performance to expectation
Performance Metrics:
The developed system is successfully performing its job by eliminating the human effort to water the plants. We have chosen three different areas on our campus to check the system performance on three different implementation methods. We implemented the system on POT LEVEL, CROP LEVEL, and GARDEN LEVEL. We get the desired results and efficient metric values with provided telemetry and computation in the Terasic DE10-Nano development board. In POT LEVEL, the system works with 94.37% efficiency, in CROP LEVEL, the system works with 97.88% efficiency and in GARDEN LEVEL, the system works with 96.14% efficiency. The system also saves the records of logs in the cloud storage point. The below attached are the saved logs sent from the primary control unit and the secondary control unit to the database.
Some other metrics:
Telemetry sent from the primary control unit to the cloud unit
Telemetry sent from the secondary control unit to the cloud unit
Minimum - Maximum temperature plot
Performance to expectation:
The system successfully achieved better results in the conducted trials. It is 100% Specific to the sustainability goal which is to regulate overwatering and underwatering, mentioned in the "Expected Sustainability Results" section.
The performance is 97% Measurable and is preferable to calculate the amount of water delivered.
The goal is 98% Achieved. We tried to bring it to 100% with more trials. But what was the promising parameter we understood was, that it can be brought up to above 99% if the pipeline system is perfectly designed.
The developed system is 100% Relevant to the goal mentioned. It eliminated the human intervention while watering plants and removed the dependency on more number of sensors.
The designed system achieved 94% for the Time-Bound parameter since we thought to make it more advanced within the time. We are still working on some other parameters and algorithms.
7. Sustainability results, resource savings achieved
Sustainability Results:
We tested the system in three different implementation methods mentioned above.
The pot level works fine and the performance is up to the requirement.
In crop level implementation, there is one particular plant (Eugenia) in 25 numbers and each plant takes about 1.7 liters of water /day.
Calculating the total amount of water all the plants needed in a day, 1.7 * 25 = 42.5 liters
Here, we observed that the gardeners are watering them with a container of 6.5 liters for each plant per week. The water container for every plant once per week. So, 6.5 * 25 = 162.5 liters/week (for all 25 plants).
The plant actually needs, 42.5 * 7 = 297.5 liters/week.
Making the math 297.5 - 162.5 = 135 liters/week, the plants are not getting the required amount of water.
The underwatering is observed and with our autonomous system, we supplied about 41.6 liters of water for each plant, and for all plants, we delivered about 291.2 liters of water per week. In the crop level implementation, the system delivers with around 97.88% efficiency.
In the garden level implementation, there are 5 different kinds of plants, and based on density calculation, they all need 80 liters of water per day in total. Calculating the total amount of water needed per week, 80 * 7 = 560 liters/week. The gardeners are watering all those plants with a total of 800 liters container tank every week. Calculating the math, 800 - 560 = 240 liters/week. Here the plants are overwatering and around 240 liters of water is getting wasted every time they water the plants. With our system, we delivered around 581.6 liters/week by saving around 218.4 liters of water. In this implementation, our system delivers around 96.1% of efficiency.
Resource Savings Achieved:
Resource Saving and Sustainability Results (Calculation based on implementation tests)
8. Conclusion
Can you describe why your sustainability goals are significant?
Climate action is one of the sustainable development goals proposed by UNDP. Water Conservation and Climate Change are the two major global challenges that we are facing even these days. The prominent solution to solve these issues is only to create an effective awareness among the people.
Our project’s primary goal is not just to save water. It includes regulating water waste and saving water resources by providing a conscious awareness to humans. Every plant needs water to grow. But when we supply the water with an exact measure that even though it is actually necessary for a plant to grow, the humans might understand how to utilize the resource properly. And these could be an effective way of creating awareness of conscious usage of water. And that is exactly why we believe that our sustainability goals are significant.
Is the project capable of making a real-world impact on our use of the world’s resources?
Project Green-Globe delivers the essential requirement of saving water by regulating Over-Watering and Under-Watering. Our project is actively addressing two of the major global challenges that are Water Conservation and Climate Change.
With this project, we can save water resources, and also, we can grow the plants effectively and efficiently, which creates a direct impact on Climate Change and Water Conservation. And there is N number of offices, houses and other areas where they grow gardens and water plants. So, when this system is implemented on a larger scale, the results would be more life-sustaining.
One of the major goals of our project is also to create a benchmark for the initiation of connecting the edge technology to conserve natural resources and address global challenges. With the hope by the time our project will be live, we expect more such people and most promising project ideas would come to solve major problems of nature just like our project.
Do you think your project could meaningfully reduce environmental impact or help people live better lives?
Our project promises to solve the sustainable development goal which is Climate Change and Water Conservation, as we addressed. Our project could meaningfully reduce climate change and creates an environmental impact by addressing Climate Change and saving Water resources.
Our project could also make people live better lives by automating the job of watering plants. It doesn’t mean that they’re struggling while watering the plants. But even though on the small scale, it is affecting nature when they couldn’t properly look upon plants and trees they’re supposed to look upon and grow. So, we can verdict that even if humans fail to look upon nature, automating that job could meaningfully make their lives better.
When humans became selfish and busy, nature will still provide the resources to humans. So, when nature lives better, humans will also live better. And making a project that looks upon nature will eventually benefit the people and make them live better lives.
Research papers, that quantify the issue that we are solving:
We have gone through some research works that quantify the issue we are trying to solve.
There are two journals from IEEE Xplore, which are relatable to and quantify our work.
One is the Smart water protocol for the irrigation of Urban Gardens in Smart Cities, stating about providing communication protocol for smart irrigation systems designed for Smart Cities.
https://ieeexplore.ieee.org/document/9751098
The other one is the IoT-Based Dynamic Irrigation Scheduling System for Water Management of Irrigated Crops, which addresses the water management for agriculture crops.
https://ieeexplore.ieee.org/document/9249427
There is a research work from “Aarhus University, Denmark” stating that Worldwide water shortage by 2040.
https://www.sciencedaily.com/releases/2014/07/140729093112.htm
In that, they are addressing water conservation to produce electricity. The bottom line of that research work is ultimately about utilizing the water resources meaningfully, which supports our project goals.
There is this other publication from the “United States Department of Agriculture” stating that Water Conservation in Irrigated Agriculture.
https://www.ers.usda.gov/webdocs/publications/44696/30956_eib99.pdf
In this work, they are ultimately addressing Irrigated agriculture, which accounts for 80-90% of consumptive water use in the United States.
“National Center for Biotechnology Information” also mentioned that Weather-sensitive irrigation is one of the four case studies on The Impacts of Water Conservation Strategies on Water Use.
https://www.ncbi.nlm.nih.gov/pmc/articles/PMC3307622/
Conclusion:
Project Green-Globe is a customized and intelligent watering system for horticulture. We developed and successfully implemented the authentic system for autonomous irrigation for horticulture needs. The system runs with an efficiency of around 95% and fulfilled the required needs for the performance expectation. The authentic system developed eliminated the need for manpower for delivering the water. It also reduced the dependency on sensors for irrigation management. The control units are efficient enough to perform complex logic operations making the system implemented on larger scales of gardens and crops. The control units are pushing the data to the cloud storage point to save the logs for further reference. Ultimately, the system is able to regulate the underwatering and overwatering issues helping in addressing the global challenges and conscious usage of water. We hope this initiation of connecting the technology to conserve natural resources and address global challenges, and we strongly wish our thought will move forward and more people will come forward to find the solutions for global challenges with the edge technology and create sustainability between humans and nature.
1 Comments
Please login to post a comment.
AP062
Appreciations and suggestions are always welcome!