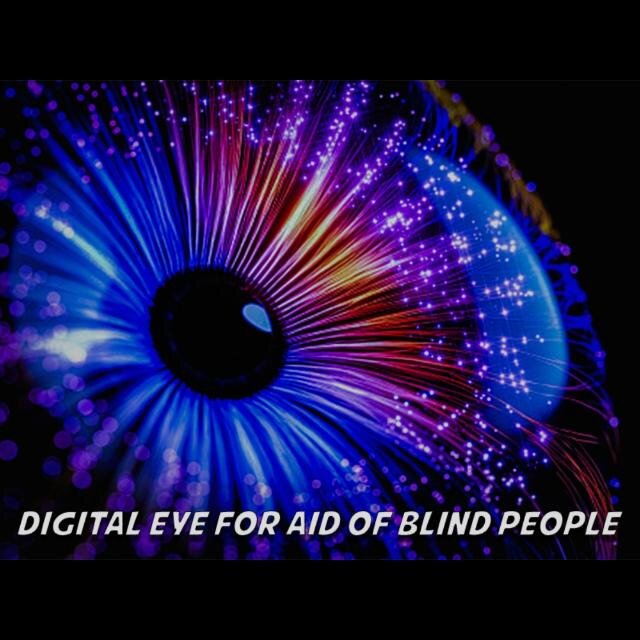
Every citizen has their basic rights to live a healthy and independent life likewise the blind and partially sighted people should lead their lives independently.
Through our project we aim to help the blind and visually impaired people which makes them independent to a certain extent. The aim is to use the FPGA and Microsoft cloud to build a prototype of the device. The idea is to guide the individual by giving the necessary instructions to reach their location and also by using image processing techniques, the device will be able to convert the text to speech which makes him/her independent to the most extent. Earlier there are projects which are aimed at obstacle avoidance using Ultrasonic sensors whose range is not good enough to roam on the roads. There are also projects which need implantation of electronic chips in the visual cortex to make the blind people see but the implantation is expensive which needs highly skilled surgeons. Our Prototype plan includes the glasses , blue tooth pen-cam and headphones.
So we want to design a cost effective and portable device which performs the 3 tasks:
1.OBSTACLE AVOIDING
2. READING THE TEXT THE DEVICE HAS CAPTURED WITH PERMISSION OF THE USER.
3. NAVIGATION SYSTEM AND SAVE DATA ABOUT THE FREQUENTLY VISITED LOCATIONS AND GUIDE THEM IN FUTURE.{ i.e the device has to be trained to save the frequently visited locations such as workplace, hospital etc.,.}
Demo Video
Project Proposal
1. High-level project introduction and performance expectation
According to the World Health Organization, around 40 million people in the world are blind, while another 250 million have some form of visual impairment. The biggest challenge for a blind person, especially the one with the complete loss of vision, is to navigate around places and another problem is braille is that not every textbook, letters ,newspaper are written in braille script format to avoid all such probblems we have come up with digital eye concept.
The purpose of this project is help the visually impaired and blind to make them indepedant to the most extent
Targeted Users:For blind and visual impairment
In Task1[Obstacle Detection ]: Earlier Obstacle detection techniques involve ultrasonic sensors, where one is able detect the object but cannot give information about the object. Image processing techniques for the object detection has far more advantages over using ultrasonic sensors such as providing info about the
object. As we want the information of the object detected, here we employ object detection and avoidance using Image Processing Techniques.
Functionality:
When the camera captures the image,we use Scikit image processing techniques to find the object type and using some other adjustments in the pen-camera we find the distance between the object and the person.As we wish to give that processed informaton to the user as audio,it is so annoying to give info about each and every object captured.So to avoid this, we can set some priority on which the info has to be passes to the user as audio.We thought of setting another priority, as we can find the distance of the object from the person, why can’t we set some distance range so that if the object is inside the distance range,we will pass the info.On the other case ,if the object is outside the distance range,even if the image is captured,we wouldn’t pass the object info to the user.The range will we 3 metres fixed but futher user can reset the distance range.The priorities are as follows,
- Obstacle
- Road Infrastructure
- Path Tracking
In Task2[text to speech conversion]: Reading the text based on user's choice
Functionality:
Here in this task we are During the picture capturing, if there is text in the image, the image processing unit detects it and asks the user for permission to read the text. If the user permits to read the text, the text will be given to the audio module (we will use python ) where there is a text to speech converter which enables the user to listen to the text the camera has been captured. In this whenever the user has to read a book or a page, the image processing unit detects the edge which gives the start of the page and the process repeats.Here Optical Character Recognition is the technology in which the Handwritten, typed or printed text into machine-encoded text. The OCR process involves five stages they are preprocessing, image segmentation, feature extraction, image classification and post-processing.
It consistes of four units:
Input unit: camera takes the input from the user
Image processiing unit: The snaps from the camera is given to FPGA image processing unit . The unit detects the type of object that is Vehicle,persons etc.
Decision Making Unit: Makes decission based on the type of detected objects. And makes instruction TO CONTROL USERS MOBILITY.
Output Unit: Output as audio(instructions) from the decissions unit about next stop to be taken by the user.
In Task3[NAVIGATION SYSTEM AND SAVE DATA ABOUT THE FREQUENTLY VISITED LOCATIONS AND GUIDE THEM IN FUTURE]:
In our daily routine to reach a certain location, we can pass through our previous known path or if the path is not known, then we will take the help of others or the GPS and navigate according
to the direction which they suggest. But for the visually impaired people, it's hard to reach a location even if it is frequently visited due to traffic, lanes, obstacles etc. And to reach a new location even though the directions are specified by GPS or others, it's very hard for them to self navigate according to the specified path directions and turning points. This task objective is to provide a guiding system for the user to direct along the specified path (including the error path detection) and to save path data about the frequent locations traveled by the user.
Functionality:
To reach a specified location first the user need to provide an instruction to the design according to the user input choice which we train to the design. And then the user need to give the name of the location in the form of speech. And the user's voice input will be received by an audio receiver. The received input will be will be converted in the form of text using speech to text conversion the text will be given as input to the GNSS system. Here we need to interface some of the IOT components such as arduino GPS datalogger ,SD card module etc. And from the GPS module we obtain a 'kml' location file from the server and this kml file is given as input to a GPS-visualizer to generate a data path coordinate file ,which will be downloaded from the visualizer and stored in a micro SD card. The coordinate file consists of a collection of data points about the latitude and longitude coordinates and altitude values taken at a certain distance rate.
GUIDANCE OF THE DESIGN:
According to the stored path coordinates,using machine learning techniques, the design will generate a regression polynomial which fits closely to the datapath. When the user starts to travel ,the GPS data logger generate a coordinate file about the user's path of travel. According to the partial stability of any of the coordinate it suggests a straight away path to travel by generating a voice-output through microphone top travel straight until a sufficient distance to take diversion or until an obstacle attained in the way.
Condition 1: Obstacle in the path
If an obstacle such as vehicles ,infrastructure etc. araised in the path then according to the instructions of task1, a certain priority of distance will be created such that, inside a specified range of distance if the obstacle is detected then the control unit of the design generates a voice out put to stop or to take diversions in order to avoid the obstacle. And also the task1 will execute simultaneously, which in-turn generates the name of the object through microphone using image processing technique.
Condition 2:Diversion in the path
If an obstacle such as vehicles ,infrastructure etc. araised in the path then according to the instructions of task1, a certain priority of distance will be created such that, inside a specified range of distance if the obstacle is detected then the control unit of the design generates a voice out put to stop or to take diversions in order to avoid the obstacle. And also the task1 will execute simultaneously, which in-turn generates the name of the object through microphone using image processing technique.
Condition3: Error in the user's path
If an obstacle such as vehicles ,infrastructure etc. araised in the path then according to the instructions of task1, a certain priority of distance will be created such that, inside a specified range of distance if the obstacle is detected then the control unit of the design generates a voice out put to stop or to take diversions in order to avoid the obstacle. And also the task1 will execute simultaneously, which in-turn generates the name of the object through microphone using image processing technique.
PATH STORAGE OF FREQUENT VISITED LOCATIONS:
As we know that the data-path of different locations are stored in the micro SD card but after reaching the location the stored memory of the SD card will be deleted under consideration limited storage of the SD card. But for frequent visited locations its quite complex to take data-path from the GNSS server every day. To avoid this the design will be trained by the locations for specific no of days. And from the locations captured in the design in training data duration, the design will segregate the frequent locations and the corresponding data-path will be stored in the SD card. Hence for these locations, the design need not to take a separate data-path but it only takes user's location and continue with the previous data-path polynomial
Task 4 [FACE DETECTION AND RECOGNITION] :
If this block is activated, then it captures the frames from the camera and by using various image processing techniques, at first the face of the person captured in the frame need to detected and set for a detection count. And simultaneously the distance of the face from the camera can be calculated using the dimensional ratio aspects of the detected face. And if the face is detected in a specified range, then the detection count will increase. Upto a maximum extent of the count, this procedure willl continues. If the count reaches to its threshold value then the face recogntion block will be activated and the last captured frame will be fed to the stored face data. If the face is matched the device immediately sends the voice output with the name of the person If not it will also be stored in the saved images. From the next iteration the save face will be updated with added face.
Task 5 [CURRENCY RECOGNITION] : :
If this block is activated, then immediately the face detection untit will activate. If any face is detected on the frame. Then the image will be sent to the face recogntion block of the cloud section and the result of prediction will be stored. And the predicted result will be compared with the faces of the national deligates on the currency and immediately the text recogntion block will activate. The result of text detection block is segmented according to the curency value priority and compared with the values of currency. And the finalized value will be sent includeing the type of curerncy to the voice outuput unit of the device.
2. Block Diagram
3. Expected sustainability results, projected resource savings
EXPECTED RESULTS:
- Guide the user (blind/visually impaired) to desired location he wants, which he inputs through the microphone to the design.
- Guide the user to take turns, and warn them if they deviate from the path that the GPS has already provided.
- The processed image information about what the user has seen (i.e., the captures of the camera) has to be delivered to the user based on the priority we have set i.e.
a) Vehicle information
b) Path information and so on....
- The design has to pass the information about surroundings to the user up to the certain limited range only (i.e., image information should be passed to the user if the distance from the object that is captured is below the present threshold for safe movement.)
- The design is supposed to recognize the text on the images, and ask for user permission whether to read it or not, if the user is willing to listen, the design reads the text so that user can listen to it through the speaker (one of the output device), else it erases from the storage.
- The user will able to recognize people based on pre-stored database stored in cloud.
REASOURCE SAVING:
The human resource plays a significant role in the economy of a country by contributing to productivity. The other resource becomes useful because of the input by the human resource.
Investment in human capital yields a return and it is done through education, trainings, and health. It is truly known that a person who is educated earns better than an uneducated person. Also, a healthy person is much more productive than an unhealthy person.
Our prototype focus on health care of a blind person. Blind person need to lead their life in a efficient and independent manner likewise the common people leading their life.
Road accidents Occurance According to (TRID)Transportation Research Information Database & (WHO)World Health Organization reports:-
a)Approximately 1.3 million people die each year as a result of road traffic crashes.
b)The United Nations General Assembly has set an ambitious target of halving the global number of deaths and injuries from road traffic crashes by 2030 (A/RES/74/299)
c)Road traffic crashes cost most countries 3% of their gross domestic product.
d)More than half of all road traffic deaths are among vulnerable road users: pedestrians, cyclists, and motorcyclists.
e)93% of the world's fatalities on the roads occur in low- and middle-income countries, even though these countries have approximately 60% of the world's vehicles.
f)Road traffic injuries are the leading cause of death for children and young adults aged 5-29 years.
Iilliteracy rates of Visually Impaired person in the world according to research:-
The world’s 37 million blind people, an estimated 90% live in developing countries. About 15 million of them live in India, a number that has doubled since 2007 to make it the highest number in any country. However, the braille literacy rate there is only 1%, far lower than the regular literacy rate of 77.7%. This presents a significant problem; without braille education, the quality of life for visually impaired people is significantly decreased. Without braille literacy, blind people are often unable to understand or use written communication. As a result, they require an interpreter to read and write for them, which can limit their employability.
Resource Saving Using Our Prototype:-
4. Design Introduction
FIGURE 4: THE DESIGN OF THE DEVICE IMPLEMENTATION IS AS FOLLOWS
4.1 HARDWARE IMPLEMENTATION
The processing parts of the project is completely implemented using
DE 10 nano FPGA board and the RFS card provided with it to connect to the Azure cloud. For directions of the navigation, we have used magnetometer equipped on the RFS card and on-board accelerometer to specify the rotational deviation and the rate of deviation in the user’s position.
In navigation block, we have used an USB GPS receiver (Dongler model) to attain the user’s current coordinates in the path. And to collect the coordinates of the destination location and the shortest path mapped to it, we have used Azure maps and the retrieving process of the mapped coordinates is done from the Azure cloud.
Triggering Mechanism of the device using GPIO :
For functionality of the project, we have designed a switched Triggering unit in which 5 GPIO pins are enabled as 5 unique triggers for the execution of 5 blocks. From GPIO 1803 to 1807 are considered as triggers for Navigation and vehciel avoidance block, Face detection and recognization block, Object detection and obstacle avoidance block, text detection and recognization block and finally Currency recognization block respectively. If a trigger is set to be HIGH, then the corresponding block will be activated with a certain amounts of external time limits except for navigation block.
This device prototype can work with both single block execution and multiple block execution.
Single Block Execution :
In single block execution i.e only one trigger is active HIGH and the others are LOW, then except the navigation block, each block will be active and execute for a period of 30 minutes and after that the complete block will be reactivated if the trigger is still found to be active HIGH.
Multiple Block Execution:
Priority levels of the blocks will be considered in this execution. The levels of priority of the design :
Navigation and vehicle avoidance Block (Highest prority block and with no external time limit) >
Face detection and recognization Block (Second highest priority block) >
Object detection and obstacle avoidance block (Third highest priority block) >
Text recognization block (Moderate priority block) >
Currency recognization block (Least priority block)
If two or more triggers are active HIGH, then the design will start with highest priority block and starts with the execution of each block with a limiting duration of 1 minute. Then according to the priority of the block, sequential order of the block will be choosen.
Finally in this condition, all the blocks except navigation block will acts as periodic blocks of period 1 minute.
Multiplexing Voice Unit of the Device :
Multiplexing Voice output Unit :
For the input and output instructions to the user, a microphone (Zebronics), and a speaker (for emitting the instructions in demonstration) are connected to a raspberry pi board. For this instruction block, we have created a multiplexing voice unit, in which the GPIO sections of the DE10 nano board and a Raspberry pi board are connected with a set of 5 pins. Using this multiplexing unit, upto a set of 32 combinational GPIO values can be transferred from the FPGA board to raspberry pi board. And according to the GPIO code generated, the corresponding voice output instructions of the device will be generated in the Raspberry pi section and the instructions will be emitted through a speaker from Pi board.
CONNECTIONS |
![]() |
And for some messages GPIO 24 as input trigger to send any data from the DE 10 nano board we have used serial communication using USB UART protocol and the corresponding data wil be saved and read from the voice output block and speaker.
Multiplexing voice input unit:
And a GPIO 23 pin is connected from raspberry pi board to DE10 nano board which acts as a trigger to take input instructions through the microphone from pi board and transfer the instruction to the FPGA board through serial communication.
For capturing the input snaps of image processing techniques of face detection and recognition, object detection/recognition, text detection/recognition and vehicle detections, we have used a mini usb camera (TFG SQ8 1080p mini camera) with a frame rate of 2.
4.2 SOFTWARE FLOW
Image processing section is the major software block of our project, which includes face recognition and detection, object recognition, detection and avoidance, vehicle/pedestrian detections and avoidance and finally text detection and voice generation.
In the aspect of face recognition, at first the face need to be detected. For detection of the face, we have used viola jones algorithm and multi scaling using the haar cascade classifier. If a face is detected, it sets count and if the count reaches to its maximum, the face recognition will be activated. Initially it collects the characters of the face and compares the characters with the stored image data base and returns the matched face name. If the face is not matched with any one, then the device asks for the name to save the last captured image. Based on the user’s choice the face will be saved or neglected.
In obstacle/pedestrian/vehicle detection and avoidance, first of all the image will be compared with the pre-trained models of cvlib using the tensorflow and the detected object will be given a rectangular box of detection. And then the detected object will be classified for vehicles/pedestrians and then using the decision making algorithm will tend to instruct the user to navigate along an appropriate direction.
In the aspect of optical character recognition, the image will be color mapped from RGB to HSV and then every character in the image will be detected and recognized using py-tesseract algorithm. And then the complete text will be emitted in the form of voice output word by word.
In the navigation block, the current coordinates from the user will be fetched from the USB GPS dongler. And then from the microphone the device will take the destination name from the user and then the user it maps shortest path coordinates from the current location to the destination. And then by calculating the angle and distance between the coordinates, the device unit compares the angle with the magnetometer reading of the RFS card and guides the user to travel in an appropriate direction.
And finally the Currency detection block is implemented on a basic algorithm based on face recogntion unit and text detection unit. When this block is activated, and if the front portion of the currency note is captured,it will be sent to the face detection block. And if the face is detected on the note then immediately it sets face recognition block to identify the face of the person in the list of national leaders on the currency. And based on the detected leader, the cooresponding country is detected and immediately the text detection block gets activated. And if the initial line of detection contains the currency value, then the device generates a voice output to the user with type of currency and value of currency.
5. Functional description and implementation
5.1 FACE DETECTION AND RECOGNITION
The complete implementation of the face recognition unit is processed under the Azure cloud video processing sections in which the huge models of the saved faces can be saved in the containers created in it. For face recognition, at first the detection block should be activated. The process of face detection is completely based on the viola jones algorithm in which a numerous images are collected and compared for different characters of the face such as the ratio of the facial elements, lengths and widths of the parts of the faces etc. and are tuned to generate a Haar Cascade classifier in the xml formal. The generated classifier is trained to apply for the multi-scaling operations on a gray scale image to notify the detected segments of the face from the input image.
To activate our face recognition model, we used this face detection as a trigger such that, if a face is detected more than a limited number of times under the consideration of a distance range. Then the last captured face is stored and sent to the face recognition block of the cloud unit. There it compares the facial characterstics of the sent face with all other faces stored in the database and then the name of the matched face will be returned to the user in the form of voice output
5.1.1 Face Detection & Distance Measurement
Image is captured by the camera.
5.1.1.1 Input Frame
The image captured is given as input to the device prototype.
5.1.1.2 Gray Scale Image
The input image is processed and converted to Gray Scale.
5.1.1.3 Output Frame
The output frame with the detected face and distance from the user is printed on the frame.
5.1.2 Face Recognition
5.1.2.1 Input Frame
5.1.2.2 Gray Scale Image
Input frame is converted to Gray Scale Image for further processing.
5.1.2.3 Output Frame
5.2 OBJECT DETECTION AND RECOGNITION
The object detection section also simultaneously run under the processing sections of the Azure cloud in which the base modules of tensor flow and pre-trained models of cvlib are stored. In the block of object detection, first the captured image will be color mapped from RGB to BGR and then compared with pre-trained models of cvlib.Then the detected image will mapped with boxes with the detection label. According to the type of detection label, it makes decision to activate obstacle avoidance block or not.
5.2.1 Input Frame for Object Detection
5.2.2 Output Frame for Object Detection
5.3 OBSTACLE AVOIDANCE
Trigger to the obstacle avoidance block is given from the object detection block, if the collected image is classified as a vehicle/pedestrian then , then design will convert the frame into different image processing techniques to calculate the density captured in the image and makes appropriate decision to move in a specific direction.
5.3.1 Input Frame for Obstacle Detection
5.3.2 Output Frame for Obstacle Detection
5.3.2 Guiding Appropriate Direction to Avoid Collision
5.4 OPTICAL CHARACTER RECOGNITION
For aspect of optical character recognition, the image will be color mapped from RGB to HSV and then every character in the image will be detected and recognized using py-tesseract algorithm. And then the complete text will be emitted in the form of voice output word by word.
5.4.1 Input frame
5.4.2 BGR to HSV Color Conversion
5.4.3 Text Detection
5.4.3 Text Reading
5.5 NAVIGATION OF THE USER TO THE DESIRED LOCATION
The navigation block is completely implemented on the HPS section of the DE10 nano board. The Navigation starts from the user giving the destination location through audio. When the user inputs the destination location, the corresponding co-ordinates of entire path from the user’s current location to destination location are fetched using Azure Maps. A GPS tracker is attached to user which fetches the current location i.e. current coordinates of the user. The algorithm compares the co-ordinate from the Azure Maps and the current location and give appropriate commands using octa segment segregation of the coordinates of all directions. And the execution of obstacle/vehicle/pedestrian avoidance for the user for safely reaching the destination.
Meanwhile, during the travel across the path, if the user encounters any obstacle, the system guides the user accordingly using collision avoidance algorithm.
5.5.1 Path Mapping from Co-ordinates collected from the Cloud
5.1.1.1 Co-ordinates from the cloud
Test run on Postman API Tester for Azure Maps.
A request is sent to the Azure Maps requesting the co-ordinates from source and destination locations. The tester gives some of the co-ordinates along the path from the source to the destination. An algorithm developed to map those co-ordinates using regression mapping.
Fig. Requesting the Azure Maps API
Fig. Co-ordinates from the API
5.5.1.2 Maps Generation from the above Co-ordinates
5.5.1.3 Original Map from Google Maps for Comparision
5.5.1.4 Commands to the User According to the Map
5.6 Currency Detection and Recognition
- 1. This is a basic currency recogntion block which is completely dependent on the face recogntion and text recogntion algorithms.
- 2. If the trigger for this block is activated, then immediately the face detection unit will activate. If any face is detected on the frame,
- 3. Then the image will be sent to the face recogntion block of the cloud section and the result of prediction will be stored.
- 4. And the predicted result will be compared with the faces of the national deligates on the currency and immediately the text recogntion block will activate.
- 5. The result of text detection block is segmented according to the curency value priority and compared with the values of currency.
- 6. And the finalized value will be sent includeing the type of curerncy to the voice outuput unit of the device.
- 7. Finally if the duration of the block is excced than the required duraion, then block will terminate.
5.6.1 Input Frames for Current Recognition
5.6.2 Output Frames With Detected Currency and Recognition of Amount of the Currency
6. Performance metrics, performance to expectation
7. Sustainability results, resource savings achieved
HOW FAR OUR PROTOTYPE REACHES SUSTAINABILITY GOALS:
Real - time Prototype Test:
We have tested our prototype device on a total of 16 Blind people in which 9 poeple are students and 5 people are middle aged persons and the remaining two people are old people.
From the feed back of device trail on the students, It is found that text recognization is working with clear audio demonstration of the text with pyttsx3 module and also with good accuracy levles of detection. And the real time object detection is also very helpful to know the object in advance to recognize/ And in case of obstacles it is perfectly deviating the user from the obstacle.
We have tested our navigation block of the prototype on middle aged persons. which have accurately mapped the shortest path from the person to Destination location and navigated along the path. He found 4 cars and 11 bikes on the road. The device has detected 4 cars and upto 10 bikes in advance, when they are upto a distance of 6 meters from the user and guided according to the frame of coverage of the vehicle. From this device test, we have found that the navigation block is working with above 90% and the vehciile avoidance block is working upto 85 to 90%
And the face detection recognition block and the curerncy recognition block is tested for all the blind people. If any person is inside their range limit the face is getting recognized and some faces were asked with the name to save the person name. It has worked perfectly above 90% and the blind people are easily recognizing the people in the saved images.
IMPACT OF OUR PROJECT ON ROAD ACCIDENT DEATHS:
-> Most of the blind persons who are travelling on the roads are suffering a lot from the heavy traffic on the roads which are not abling the blind to cross the over roads or to travel along a path. In search of path most of the blind people are are crossing over the vehicles and many of them are died due to this probelm. But our project can give a conclusion for this probelm.
-> In the navigation block of our design, If any vehicle and pedestrian or potholes are detected on the road then, our prototype will detect them in advance using distance priority which is very helpful in preventing road accident deaths of blind people.
[around 30-40% blind person are leading to death or some injuries due to disability]
BLIND ASSISSTANCE OF THE PROJECT:
-> The human dependency in navigation and assissting in various situations can be eliminated by using our project. The user can independently travel to the any location where he want to reach. The user can independently detect hsi rquired books or articles and he can read. He can independently recognize a person and make a conversation using the assistance system of our project.
-> And many visually impaired persons are loosing their confidence to live with out proper sight of vision. So most of them are committing suicides despite of their disability. Our project will gives a proper guidance system and provides a better hope to the blind people to live independtly.
IMPACT OF OUR PROJECT ON BLIND STUDENT’S LIFE
-> Till now the Braille script is the only way of blind people to study the letters and different forms of text. But the Braille script is not encrypted for all kinds text in our day to day life such as news papers,, which is an impossible task for a blind student to study. Here our prototype is much useful to recognize any type text imprinted anywhere.
-> Even though the blind schools and institutions are educating the students, the students need to suffer with a lot of problems to study and analyze the educational concepts. Using our project they can easily visualize many objects or images and can read any type of text, to have better educational life than the past.
-> By using our project with out depending on any one, the student can reach his school/college from the fetched and stored coordinates of the school/college and can detect books and the names of the books by his own. He can recognize his friends, classmates and teachers. He can detect his daily routine objects and can egage in his usual activities faster than the past.
IMPACT ON REAL-TIME PROBLEMS
-> Reaching a location for a blocind person is really a difficult task. But just based on the destination location name the user can travel to any known or unknown location and the user can be protected from the vehicles or potholes due to the guidance of the device to deviate.
-> Thus this project helps us to avoid many road accidnet deaths of blind people and even if the the user's path is deviated from the original path due to the high traffic, then from the user's coordinate another path will be mapped to the shortest disance coordinate of the map eith user's location which acts a path correction algorithm and guides the user to a perfect destination.
-> Many people can easily cheat the blind in the aspect of currency. But this project can eliminate the problem of money frodulency toward blind easily, since he can easily recognize the currency type and its value.
-> Many blind people usually collide to different obstacles and gets injured frequently. But this problem can also be avoided by using our project since the object can detected and segregated as obstacle and avoid them in advance.
-> The object recognization block will saves the time of the user inorder to search for essential daily needs of the user.
-> Recognizing a person for a blind person is a hard thing. By using our project, the user can recognize his family members and friends accurately and in cas of any tresspassings the device detects that a person is tresspassing into their home
-> Studying non Braille formatted books or news paper is challenging task for a blind person cand can be easily recognized and read by the device. Not only for study, but also to detect the boardings of an infrastructures or public monuments and their names will helos the user to identify the his current location
Achievements of the design:
In the navigation block the directions to the user according to the deviations angle of the user , are perfectly generated and guided.
In face detection and recognition unit, the faces are successfully detected and recognized with a greater accuracy levels
In obstacle avoidance block, the user is guided in advance and avoided from the vehicles/pedestrians.
And finally in text detection and recognition block, the device successfully generated the voice output according to the user’s choice.
Till now there are no such device prototypes which enables the user to have these many features including the face recognition models.
This prototype is a dynamic device in which the de10 nano and the Cloud segments of the design are perfectly used wit image processing and can act as a next step in sustainability development using FPGA.
This prototype can be implemented in a much portable way if the device is fabricated and equipped along with the spectacles and the headphones.
8. Conclusion
Digital eye for aid of blind people which has been designed to make a visually impaired person independent.
By making a prototype design we have achieved all solutions using Only FPGA board and Azure Cloud for all the basic problems like Making person to navigate independently, to enable user to read all types of readings, making the user to recognize persons like friends, family members, etc of the visually impaired person, real time object detecting and recognition.
0 Comments
Please login to post a comment.