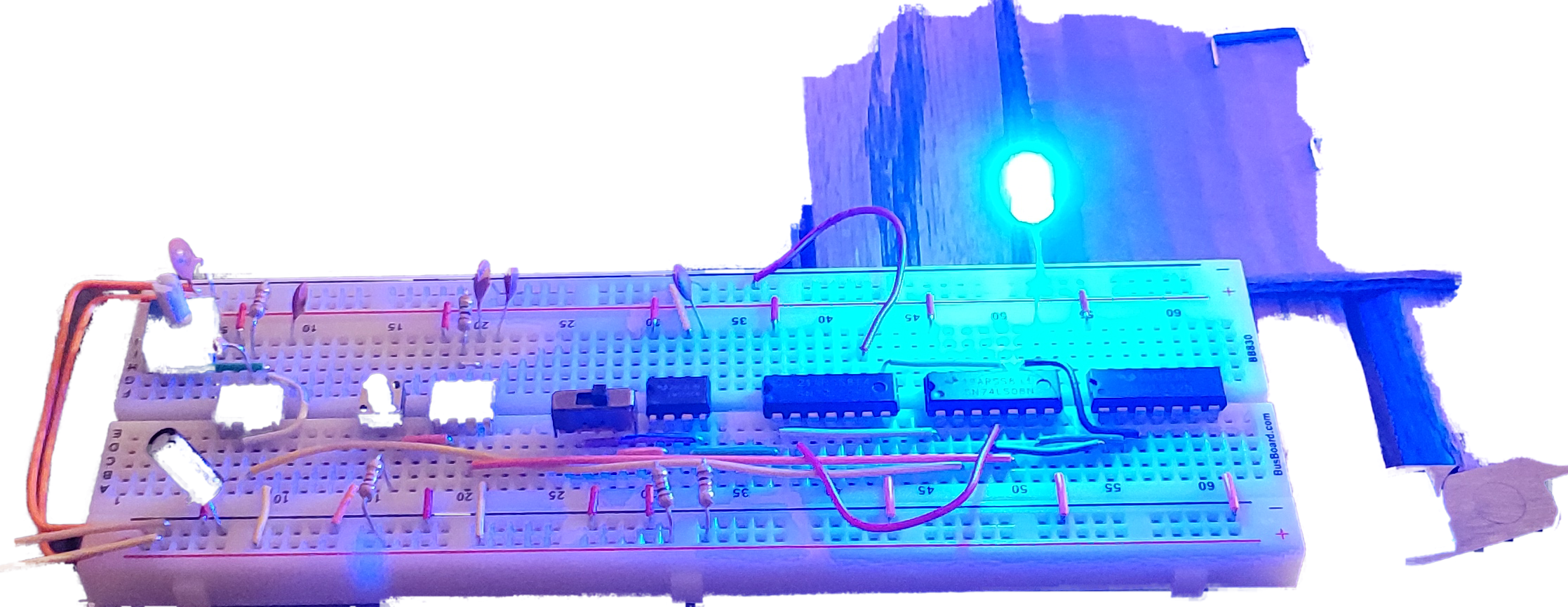
To be able to determine with a higher likelihood when to be be able to turn off electronics, lights, set air conditioning higher and not to interrupt someone at home simultaneously.
Project Proposal
1. High-level project introduction and performance expectation
This project is based on the ability to use Intel FPGAs and IOT technology to create a smart off sensor. This sensor will be important to the world because too many people leave the power on. It only makes sense to connect to new technology and give the same technique another try.
It appears that with the proper setup such as the one we will utilize that people will use programmable methods and turn off their power and water when they are not home or not in use. This detector should do exactly that. Hopefully California's will utilize this as we are now in an emergency power and water situation.
2. Block Diagram
3. Expected sustainability results, projected resource savings
This project has to be able to successfully cut power and water off. Water should be reduced by 99.5% and Electricity should be reduced by 99.9% as differentiated by switching off.
0 Comments
Please login to post a comment.