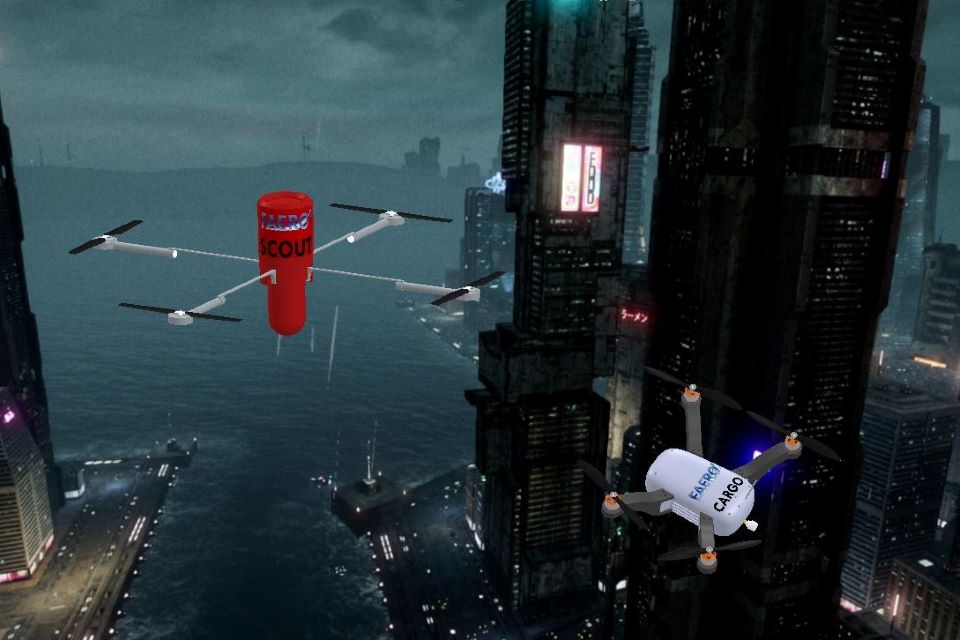
We believe the wide adoption of drones to replace current CO2 emitting delivery vans will contribute to a significant reduction of carbon additions to the atmosphere. However, if drones are not able to be adopted safely this valuable reduction of carbon emissions will not be realized. Our project combines the technologies of an FPGA, embedded processor, analog sensors and cloud communications to enable the wide and safe adoption of drones to replace traditional CO2 emitting delivery systems.
We will demonstrate an FPGA + Processor + Sensor technology that enables a scout drone to detect atmospheric upsets such as turbulence generated around buildings in windy conditions or city thermals. Atmospheric upsets of drones can cause drone loss of control (LOC), collisions between drones, and collisions with buildings or people. The scout demonstration will send near real-time turbulence location data via the cloud to cargo drones to ensure safe delivery of packages with a reduced hazard to third parties.
The key technology of turbulence and upset detection for prevention of LOC has already been developed by Foale Aerospace Inc and has been developed as a solar powered sensor system that can be attached to flying vehicles without aircraft wiring or integration. We were awarded 3rd prize by the Experimental Aircraft Association Founders Prize competition to produce solutions to prevent Loss of Control, by expert judges at Air Venture 2021 at Oshkosh, Wisconsin.
We propose to add FPGA signal processing to improve performance, reduce detection times and reduce false positive signals from our system. We propose a cloud interface will allow near real-time (a few seconds latency) hazardous conditions detected by a light scout drone to change the flight path of a cargo drone and prevent a hazardous or unsafe outcome.
We have experience in Verilog, Quartus and Modelsim targeting a Terasic DE0-Nano with a Raspberry Pi Zero processor interface written in Python, as well as Yosys/Arachne-pnr/IceStorm toolchain for Trenz Icezero iCE40 FPGA. We have 30 years of programming experience with C and C++. We will use this experience to breadboard a flight system onto the Terasic-Intel-Analog Devices-Microsoft InnovateFPGA platform. Real flight data recorded during light drone aircraft flights in turbulent and calm conditions will be used to demonstrate the identification of atmospheric conditions that cause aircraft upsets using the InnovateFPGA platform as if it were mounted on a scout drone. Communication hazard bulletins via the cloud to the cargo drone will be demonstrated via a wi-fi link to a raspberry pi based processor mounted on a wheeled rover, to demonstrate hazard bulletin reception and responsive action by a cargo drone in flight.
Demo Video
Project Proposal
1. High-level project introduction and performance expectation
Drone package delivery safety in turbulent atmosphere conditions in confined areas like Cities
See our presentation video at https://www.youtube.com/watch?v=mjRCBjk6Ixs
See our demonstration video at https://www.youtube.com/watch?v=VbMiAuBnGYQ
See our project files at https://github.com/cfoale/innovateFPGA2022-AS038
Foale Aerospace Inc Team AS038 members:
Colin Michael Foale |
Ian Foale |
High-level project introduction and performance expectationlevel project introduction and performance expectation
Figure 1: Illustration showing Scout operational deployment preceding a cargo drone with valuable cargo. When the Scout detects hazardous conditions, it causes the cargo to be saved.
We believe the wide adoption of renewable powered drones to replace current CO2 emitting delivery methods will contribute to a significant reduction of carbon additions to the atmosphere. ' Last minute' point to point courier delivery in cities predominantly relies on CO2 emitting services. These services can be replaced by solar powered drones standing by ready to serve. If all the current package delivery methods are replaced with renewable powered drones, more than 2 million tons of CO2 reduction per annum by 2050 could be realized. However, if drones are not able to be adopted safely this valuable reduction of carbon emissions will not be possible.
Our project combines the technologies of the DE10-Nano FPGA, HPS embedded processor, Analog Devices sensors and Microsoft Cloud communications to enable the wide and safe adoption of drones to replace traditional CO2 emitting delivery systems.
We demonstrate a technology that enables a scout drone to detect atmospheric upsets such as turbulence generated around buildings in windy conditions or city thermals. Atmospheric upsets of drones can cause drone loss of control (LOC), collisions between drones, and collisions with buildings or people. Our scout drone hazard detection demonstration shows how near real-time turbulence location data can be detected, processed, and interpreted for distribution via the Microsoft Cloud to cargo drones, to ensure safe delivery of packages with a reduced hazard to third parties.
The key technology of turbulence and upset detection for prevention of LOC has already been demonstrated by us and has been developed as a solar powered sensor system that can be attached to large flying vehicles without aircraft wiring or integration. We were awarded 3rd prize by the Experimental Aircraft Association Founders Prize competition to produce solutions to prevent Loss of Control, by expert judges at Air Venture 2021 at Oshkosh, Wisconsin.
The technology relied on data collection and Digital Signal Processing (DSP) running between each new data measurement, on an ARM processor. We realized an FPGA could provide concurrent DSP assessments without slowing the data flow. This feature is highly desirable to reliably detect abrupt environment hazards. We have developed FPGA based DSP to improve performance, reduce detection times and reduce false positive signals from our system. The use of Digital Signal Processing without data gaps requires the use of concurrent Hardware Description Language running on an FPGA, such as the DE10-Nano.
We use the DE10-Nano Cyclone V FPGA to fetch and correlate data with a sufficient time history for LOC identification and prediction using Verilog HDL on the FPGA. In a pre-operations mode a longer data time history is collected and saved for analysis by passing the data to the DE10-Nano HPS processor over the Intel Avalon bus interface. The longer data history is used to develop the cross-correlation targets that are used by the FPGA HDL DSP module. We use an IoT Python script running on the Linux LXDE on the HPS to pass DSP detected hazard assessments at a 1 second rate to the Microsoft Azure Cloud, which is then available to users.
This innovative FPGA based Scout drone system allows near real-time (a few seconds latency) hazardous environment conditions detected by a light scout drone to be passed to the Cloud and enable a real-time change of a cargo drone delivery, and prevent a hazardous, unsafe outcome or property destruction. When a hazardous condition is reported to the Cloud, 'Cloud Control' can issue a route change as the most likely outcome, or command a delay, similar to the way airline transport is rerouted or delayed due to bad weather. Our solution will provide drone package delivery services a reliable environment hazard detection capability, ensuring that their services can be safely, reliably, and profitably deployed.
2. Block Diagram
Block diagram
Figure 2 shows the key elements of our Scout Hazard Detection System. The system consists of:
DE10-Nano, utilizing the Cyclone V FPGA for data collection and DSP.
DE10-Nano Analog to Digital Conversion (ADC) of Scout sensor data to the FPGA.
A Scout drone sensor measuring accelerations, rotations and dynamic pressure differences caused by atmospheric flight disturbances or turbulence.
A DE10-Nano HPD Python script implementing Microsoft Azure IoT interface to the Microsoft Azure Cloud.
Analog Devices DC2025A-A Digital to Analog Converter (DAC) for data play back to the DE10-Nano FPGA ADC for DSP hazard detection assessment.
A table-top turbulent atmosphere simulator to provide flight-like physical input to the Scout drone sensor assembly, as if it were mounted on a scout drone. The simulator reproduces real flight data recorded during glider flights in turbulent hazardous atmospheric conditions.
These components can be repackaged to meet the physical and flight requirements of a Scout drone. Two different use cases are shown, with emphasis to be applied to Autonomous vehicles and Transportation to achieve the best sustainability improvements for global CO2 reduction.
Figure 2: Scout Hazard Detection System composition, realization, and exploitation
3. Expected sustainability results, projected resource savings
Expected sustainability results, projected resource savings
A 1Kg package transported 1 km by a modern truck emits up to 0.15 g of CO2. Reference https://timeforchange.org/co2-emissions-for-shipping-of-goods/ . The upstream production and delivery CO2 cost per gallon of fuel at the pump is an extra 34%. Reference https://www.sierraclub.org/sierra/ask-mr-green/hey-mr-green-how-much-co2-generated-producing-and-transporting-gallon-gas. In the US in 2020 Amazon delivered 4 billion packages, with a negligible fraction delivered using renewable energy vehicles. Total package delivery by all services was 20 billion packages in the US. Reference https://www.modernretail.co/platforms/amazon-now-ships-more-parcels-than-fedex/. Looking at the reference, demand for next day deliveries within cities is increasing steadily, and in two years it has doubled. CO2 added to the atmosphere today, by all existing package delivery services in the US assuming an average 'last mile' trip of 10 km is roughly 80 million kilograms of CO2 per annum. The US GDP is roughly 14% of worldwide GDP, or 7 times the CO2 produced in the US. In 2022 the global CO2 production caused by 'last mile' delivery services could be 7 times that of the US, 560 million kilograms. By 2050 at least a growth factor of 4 could be expected, meaning a worldwide CO2 production of more than 2 million tons per annum. If drone package delivery can become safe and available, the current use of trucks can be replaced by drone delivery using electricity generated by wind and solar systems. This will not be possible if drone delivery is unsafe or unreliable.
We show significant hazard identification of wind gusts, downdrafts and updrafts acting on a Scout drone. This will encourage drone manufacturers for package delivery to incorporate this capability into their products. We expect the Federal Aviation Administration, and the International Civil Aviation Organization will require this capability in addition to transponder and other traffic control capabilities for drones. The FAA and NASA have airspace improvement studies underway to address drone safety. Our use of FPGA and embedded system technology will introduce a new weather and hazard avoidance capability to future planning for safe and efficient drone operations contributing to the wide adoption of package delivery by drones.
4. Design Introduction
Design Introduction
Our design was inspired by the comments of a competition judge who suggested we apply our entry to drones. The event was the Experimental Aircraft Association Founder’s Innovation Loss of Control competition, held at Oshkosh, Wisconsin in 2021. Our entry received 3rd place.
The prior design for aircraft provided only moderate hazard detection reliability
Our design, called Solar Pilot Guard (SPG), employed sensors to measure instantaneous accelerations, rotations, and air stream dynamic pressures about the aircraft to detect atmospheric motions that lead to aircraft upset and loss of control. Figure 3 shows SPG wing and tail sensor assemblies.
Figure 3: Design precursor: Solar Pilot Guard (SPG).
Strong or abrupt changes in air motions can cause any flying vehicle, including drones, to change their direction of flight, resulting in loss of control, or collision with the ground, structures or worse, people. The air motions are turbulent, driven primarily by vortices that result from winds passing over or between obstacles, such as tall buildings, geographic features, or changes in local temperature.
|
Figure 4: SPG aerodynamic data is correlated to indicate an upset
The SPG used only a moderately reliable upset detection method, which compared changes in measured values between the current measurement and the preceding measurement of angle of attack alpha (dPy), the change in vertical acceleration Az, the change in roll rate Wx and tail acceleration Az. These differences were simply multiplied to produce a the simplest possible correlation, shown as Upset Roll in figure 4.
A Scout drone hazard detection system demands very high reliability.
The focus of this project is to allow a relatively light, possibly expendable, cheap drone become a reliable pathfinder scout to detect hazardous flight conditions and relay these observations to high value, less maneuverable cargo carrying drones, so that the dangerous conditions can be avoided.
There are several requirements the Scout drone system must meet. Some of these Scout drone requirements are:
1. The Scout drone and sensor assembly must be light enough to respond and recover quickly from turbulent upsets.
2. The Scout sensors must be able to detect the upsets in a fraction of a second, before the drone physically changes its motions. This implies aerodynamic forces, accelerations must be measured, before they integrate over time to become motions and changes in flight trajectory.
3. All hazardous upset motions should be reported, i.e. false negatives (missed detections) should be minimized.
4. Only upsets which are hazardous should be reported, i.e. there should a minimum number of false positive hazardous event annunciations.
To meet the requirements 3 and 4, the DE10-Nano is a good candidate because it combines both an FPGA running concurrent functions, and a processor (HPS) that can manage configuration, measurement recording and communications to the Cloud. The FPGA is used to meet requirement 3 at a constant and fast clock rate, by performing concurrent measurements and cross-correlations of multiple time points, to providing a continuous output of hazard detection level. Requirement 4 is met by using the HPS to record long time history measurements to produce a high reliability DSP target for the FPGA.
A prototype quad-copter drone configuration with a DE10-Nano mounted near the center of gravity, and the sensor assembly outboard is shown in figure 5. Not shown, but required are flexible tubes leading from the ports at the locations of the straight blue arrows, to each differential pressure sensor respectively.
Figure 5: A prototype design for a tri-copter Scout drone with the relative locations of the sensor assembly and DE10-Nano about the center of gravity. Aerodynamic upsets are shown as blue arrows pointing to ports, which connect differential pressure sensors at dPx, dPy, dPz. Resultant accelerations Ax, Ay, Ax, with developed resultant angular rates Wy and Wz are shown.
The drone sensor assembly is shown in figure 6. Although the DE10-Nano has an accelerometer, it needs to be located near the center of gravity, while the Scout sensor assembly needs to be mounted away from the drone center of gravity, to sense simultaneously acceleration caused by a differential air flow rolling the drone, correlated with a change in differential dynamic pressure. The rate gyro sensors determine the integrated effect of the initial change in forces on the drone about the center of gravity.
Figure 6: The Scout sensor assembly components mounted to provide orthogonal measurement of 3 accelerations, 2 rotation rates. Three differential pressure modules are present, connected by flexible tubing to opposing, orthogonal pressure ports, shown in figure 5.
The DE10-Nano HDL, software and data flow is depicted in Figure 7. This is accomplished using the Analog Devices DC2025A-A DAC. Although two separate DE10-Nano’s are shown, the processing capability of the HPS Linux LXDE system is sufficient to incorporate all the twin functionality into just the Scout DE10-Nano. A single DE10-Nano was the configuration used, and the DAC data presentation runs independently of the Scout DSP and IoT functionality. This provided a great capability to refine the Scout DSP HDL system with “real” data during development and verification using Intel Quartus Prime.
Figure 7: The Scout DE10-Nano HDL, software and data flow is shown, with separate functionality for the Scout and the Scout digital twin. The functionality was combined onto just one DE10-Nano platform.
The great advantage of the dual FPGA and HPS in the DE10-Nano
The DE10-Nano provided great capability to accomplish our design. The FPGA allows real-time concurrent data processing, at very high clock rates, even though the timescales of the sensor measurements did not require a PLL clock greater than the minimum 6.25Mhz provided by the Cyclone V. The DSP was accomplished easily using 49% of the DSP blocks available, leaving ample margin for further capabilities to be designed. For a different application requiring a much faster hazard detection response, simply increasing the PLL clock would suffice.
The Avalon bus interface between the Cyclone V and the HPS is so fast, that we were able to initially develop the DSP using a software program on the HPS, fetching data in batches from the FPGA. However, HPS DSP processing was not concurrent with the real-time measurements, which points to the advantages of the FPGA doing DSP rather than the HPS.
Finally, the HPS Linux Ubuntu platform was very capable to run the C programming required to capture and save data from the FPGA, for DSP development, and digital twin data reproduction and presentation using the Analog Devices DC2025A-A. The Ubuntu Linux supported the Python 3.7 Microsoft Azure IoT hub software and cloud communications.
5. Functional description and implementation
Functional description and implementation
The function of our design is to perform a cross-correlation of appropriate measured parameters in real-time, and to communicate the presence or not of a target filter to the Microsoft Azure cloud.
To accomplish this, the design implemented the following:
Gather 8 channels of data at a constant, high rate, via the DE10-Nano ADC using the SPI protocol of the LTC2668, using the FPGA and Verilog HDL.
Hold a time history of 8 channels in a first in first out (FIFO) buffer using the FPGA and Avalon HDL, for periodic transfer to HPS C program and subsequent offline data analysis.
Run concurrent DSP cross-correlation of 6 channels with a target filter using the FPGA DSP blocks.
Avalon HDL sends the result of the FPGA DSP to the HPS memory map for access and transmittal by Microsoft IoT Hub Python to the Azure Cloud.
HPS C program playback of 8 channel data to Analog Devices DC20225A-A via SPI.
DSP cross-correlation method
The channel numbering to the ADC 2x5 connector is shown in Table 1, together with the equation definition of correlation of two variables. The DSP implemented treats products of one channel value with another at the same time as x and y. Target correlation is calculated using 3 pairs of channel products as x, and the target filter values as y.
|
|
Table 1: ADC channel assignments with cross-correlation equation substituting different channels for x and y.
Design Architecture
The combined HDL and software module architecture is shown in Figure 8. Each squared rectangle represents a Verilog module for the FPGA, and a C function in the HPS. Declared Verilog wires between the modules are labeled. Data paths from the rounded rectangles are busses.
Figure 8: Design architecture. The FPGA components are shown above the dotted line, with data flow to the HPS below the dotted line using the Avalon Memory Mapped Slave interface.
FPGA module descriptions
The top Verilog module is DE10_NANO_SoC_GHRD.v.
Module pll.v is Intel Quartus IP, and reduces the 50Mhz clock to 6.25Mhz, with a subsequent division by 16 using a sub-module to 0.39Mhz.
Module adc_ltc2308.v is the SPI interface to the LTC2668 ADC. Each read is every 243us, and 1.9ms to obtain an 8-channel frame. It is controlled by signals from adc_ch_ctrl.v
Module adc_ch_ctrl.v is a state machine which controls the shifting of data into the FIFO and channel number management.
Module adc_fifo.v is Intel Quartus IP providing 8192 ADC words of storage as an asynchronous FIFO, which can be read out by module hps_read_fifo.v.
Module adc_dsp.v has a custom FIFO buffer which is concurrently accessed to use the last 5 time values of each channel and 5 target filter values to perform the cross-correlation sum with the target filter. It sets fpga_dsp_byte which is latched for 1.5s as a value of 0xFF if the hazard target is identified (value above a threshold), or unlatches and sets 0x7B if no target is currently detected. The cross-correlation products are accomplished using 18 x 19 bit words for multiplication of pairs of channel counts, and the 37 bit product is shifted 6 bits to the right to prevent overflow. The calculated DSP output is a signed 32 bit word.
Module hps_read_fifo can receive a request from HPS program hps_adc_dsp.c to read out the 8192 FIFO words over the Avalon interface. During the read out, adc_ch_ctrl.v pauses ADC reads by adc_ltc2308.v. Read out clocks are provided by hps_adc_dsp.c.
HPS programs running Terasic Linus LXDE Ubuntu 18.
Program hps_adc_dsp.c has two modes, controlled by SW[3]. Mode SW[3]== 0, monitor signal fpga_dsp_byte to Python IoT Azure, or Mode SW[3]== 1 read out 8192 words for HPS DSP cross-correlation using function hps_cross_correlate with a 16 value target filter, and saving as a datafile. This mode can be repeated continuously every 1.5s, or alternatively just once, before saving a time and date named datafile.
Program “python3.7 -u /home/root/azure-python/modules/RfsModule/main1.py” is run as a separate process, sending fpga_dsp_byte as telemetry to a Microsoft Azure IoT hub subscription, at a 1 second rate.
Program adc_dac.c reads a list of previously saved datafiles and sequentially reads out each frame to the DC2025A-A DAC every 1.9ms. The program can run as an independent Linux process during the hps_adc_dsp.c process.
Intel Quartus 18.1 Light was used for design implementation
ModelSim, Quartus Prime and Intel SoC FPGA Embedded command line tool on Cygwin were used, running on Windows 10. We did not upgrade to Quartus 20.1 because of difficulties using the Embedded command line tool on Windows Linux. A representative ModelSim wave view at the beginning of FIFO read out is shown in Figure 9.
Figure 9. ModelSim view of FIFO readout.
Design Functional Testing
The development test setup is shown in Figure 10. Testing begins with the Quartus Programmer tool loading the FPGA with the DE10_NANO_SoC_GHRD.sof. The FPGA is verified to be reading ADC data by moving SW[3] to the off position. The FPGA LED’s show the value of the channel pointed to by SW[2:0]. To read out FIFO data to a datafile, SW[3] is set on, and when readout is desired, for example during activation of the atmosphere simulator, the FPGA KEY[1] is pressed.
Figure 10. Development test setup. The atmosphere upset simulator is shown bottom left, which provides simultaneous pressure, acceleration, and rotation rate physical actions to the sensor assembly via flexible tubing, a variable pressure manifold, and an expanding bladder. The DE10-Nano ADC2x5 is shown connected to the sensor assembly ribbon cable. The Analog Devices DC2025A-a is shown currently disconnected from the ADC2x5. The plot is the HPS DSP result using an FPGA FIFO of size 256.
Hazardous DSP target filter creation using a “Hazardous” data file
Figure 11a shows the notional “Bad” Scout drone hazardous event to be detected, which was used to produce the target filter. The data file has features similar to real flight data obtained during atmospheric upsets using the SPG system described above. When real drone data during an atmospheric upset becomes available, the same DSP target production method can be used. The software program Mathematica was used to identify a target filter consisting of 5 and 16 points. First, the data is plotted in Figure 11a, then the zero signal value is subtracted from each signal, and 3 pairs of channel products with significant contributions are shown in figure 11b.
Figure 11a |
Figure 11b |
We identified the time range 400 to 525 as having the largest cross channel products, in Figure 12a and 12b.
Figure 12a |
Figure 12b |
The three product pairs are multiplied and summed to produce a correlation plot, using a 16 point time history, shown in Figure 13a. The correlation in the range 430 – 446 is taken to be the target filter defined by the values of the 16 points.
Figure 13a |
Figure 13b – target filter |
The result of correlating the 16 and 5 points target filter with the original data is shown in Figure 14a, and the filters overlayed on the original data in Figure 14b.
Figure 14a |
Figure 14b – target filter |
The 16 point filter correlation magnitude is roughly 3 times that of the 5 point filter. The hps_adc_dsp.c cross-correlation target filter function uses the 16 point filter and the FPGA DSP uses 5 points to conserve DSP blocks used in the FPGA. A target threshold of 15 is used by the hps_adc_dsp.c function to identify hazardous signals. The FPGA DSP module adc_dsp.v multiplications are not floating point, but 37 bit signed products shifted right by 6, and sums, using FPGA DSP blocks to produce a 32 bit output. The FPGA appropriate hazard detection threshold is built in by default to be 190,000 for hazard signal detection, but the threshold can be changed by running hps_adc_dsp.c to set a different FPGA value using the Avalon interface.
After the target filter was determined, the FPGA FIFO size was reduced to 256 words, 32 time points of 8 channel data to focus on only target filter time periods. When hps_adc_dsp.c is executed on HPS, the FIFO data immediately after a hazard detection is plotted using gnuplot on Linux LXDE. Figure 15a and b show a couple of examples of data and cross-correlation target filter output.
Figure 15a |
Figure 15b |
Figure 15a shows the signals and target filter output at different points in time for two different hazardous events. The threshold for detection is target = 15.
Microsoft Azure IoT Hub Hazardous Events communication
The DE10-Nano HPS Linux LXDE was provisioned to the Azure IoT Hub using the instructions at https://github.com/terasic/InnovateFPGA2021/blob/main/docs/PaaS-Provision.md. The FPGA DSP sets a byte, fpga_dsp_byte , which is accessed via the Avalon interface to the Linux Kernel memory map, and our modified IoT Python script, which sends telemetry to the Azure Iot Hub. Microsoft Azure offers only a 30-day period of free subscription, but in this short time we were able to demonstrate the atmospheric upset simulator capability to trigger FPGA DSP target filter recognitions of hazardous events, delivered to the Azure IoT Hub. Figure 16 show the transactions of the Python IoT script sending telemetry, and a snapshot of the telemetry received in Microsoft Azure.
|
Figure 16a shows the DE10-Nano HPS Azure IoT script monitoring the fpga_dsp_byte and sending telemetry. Figure 16b shows telemetry received at Microsoft Azure
6. Performance metrics, performance to expectation
Performance metrics, performance to expectation
Performance parameters considered important to the design:
Sensitivity – the design should detect all identified hazards. The sensitivity of the design has been demonstrated using multiple tests using the atmospheric upset simulator, and the system detects upset profiles when either one or more channels have signals relevant to an upset.
False positives – the design should be immune to false positives. The design is highly resilient to false positives. The high specificity of the DSP target filter cross correlation means that only atmosphere simulated upsets are detected, which are similar to the profile used to produce the target. The DSP filter method has been demonstrated using the Solar Pilot Guard sensor on our motor glider.
Latency – the design should detect hazards and be actionable in sufficient time. The physical environment changes at a rate roughly equal to the speed of the drone, at most 10 m/s. Atmospheric turbulence has a scale size similar to the buildings and features that produce it, to a scale of 1 m or less. The response time of the system should be at least 0.1s or less to perform target identification. The FPGA produces a DSP response in 5 x 1.9 ms = 9.5 ms, a factor of 10 better than required. The Azure IoT script runs at a nominal 1s rate, and the latency to display telemetry on the Azure web site is about 2 s, for an overall IoT latency of 3 s. If we assume the hazard warning received by the Cargo drone has the same latency, the net delay will be 6s. Cargo operations will not be as fast as 10m/s, but using that speed, this means any Cargo drone must trail the Scout drone more than 6s x 10m/s = 60m. This is a very reasonable operational constraint.
System resources – the design should be expandable. The greatest FPGA resource used by the design is the number of DSP blocks – 55 out of 112, or 49%. This allows an additional hazard target filter to be included, should a different type of atmospheric hazard be identified, in addition to the upsets already covered.
Digital Twin – the Twin should be able to reproduce real events for target hazard development. The DC2025A-A signal output was measured using a logic analyzer. The reference voltage was scaled by a constant calibration factor to match in the adc_dac.c program, the output channel voltages were reproduced to < 1% of the DE10-Nano ADC converted voltages. The DC2025A-A 5Mhz SPI transaction time was 6us, and a full 8 channel conversion was accomplished in 800us. This gave an ample sleep time margin for the program to wait before outputting the next data frame, every 1900us.
The major benefit of the FPGA to this solution was the ability to update and calculate the DSP target filter cross-correlation every measured data frame, every 1900us, independent of the HPS Azure IoT. This was not possible if the solution used only the HPS processor to do DSP. Using the HPS for DSP, the FIFO must be read out, preventing ADC data conversion during that time, causing a gap in monitoring for hazardous events.
7. Sustainability results, resource savings achieved
Sustainability results, resource savings achieved
When drone package delivery becomes safe and available, helped by our solution, the current use of trucks can be replaced by drone delivery using electricity generated by wind and solar systems. Our system will encourage drone manufacturers for package delivery to incorporate this capability into their products. We expect the Federal Aviation Administration, and the International Civil Aviation Organization will require this capability in addition to transponder and other traffic control capabilities for drones. The FAA and NASA have airspace improvement studies underway to address drone safety. Our use of FPGA and embedded system technology will introduce a new weather and hazard avoidance capability to future planning for safe and efficient drone operations contributing to the wide adoption of package delivery by drones, and a savings of more than 2 million tons of global CO2 per year in the year 2050.
We have demonstrated that our design can meet the requirements to enable safer Cargo drone deliveries using a Scout drone. When the system is realized as a large-scale operational system, the use of Cargo drone deliveries will be a reality. This will have a real-world impact on how Smart Cities deal with congestion of both CO2 emitting vehicles as well as electric vehicles in city environments.
We expect groups of drones in Smart Cities to be deployed in a similar way to electric bicycles in cities like Cambridge, England, or Berlin, for example. These small cargo drones will serve ‘last-minute’ delivery orders, replacing ground-based courier services that contribute to street congestion. The drones will be completely autonomous, solar powered, and could be stationed on balconies or the tops of buildings, waiting for an order. The convenience and low environmental impact of accessible drone services for last minute grocery orders, medicine deliveries, postal services and general local purchases will help people to live better lives.
We have a vision for the future in which resource savings and sustainability are paramount. The vision starts with an acknowledgement of the problems we face today. By 2050 Global warming and sea level rises will dramatically affect coastal cities. See the “Land projected to be below annual flood level in 2050” https://coastal.climatecentral.org. For example, major areas of London, Hong Kong/Shenzhen and New York will be below the annual flood level. We imagine these cities attempting to transition to canal-based transport, such as in Venice, Italy. Global climate change will have a significant effect on food crop production, according to NASA. See “Global Climate Change Impact on Crops Expected Within 10 Years” https://climate.nasa.gov/news/3124/global-climate-change-impact-on-crops-expected-within-10-years-nasa-study-finds/. The steady increase of CO2 is a significant contribution to the progressive warming of our atmosphere. As humanity inevitably develops new technologies and enterprises to overcome its challenges, technologists must always be thinking about reducing CO2 in the atmosphere.
We recognize that our solution can only be a small part of the global solutions required to reduce catastrophic climate change for our planet. However, we are optimistic that competitions such as InnovateFPGA will provide a greater public awareness to leverage the world’s technologists to think bigger, more globally to create sustainable, resource saving solutions for our smart cities, our industries, the production and distribution of food, for conservation enterprises and for our planet.
8. Conclusion
Conclusion
Thanks to the innovative vision of the InnovateFPGA team, we have been given the opportunity to create a solution that addresses the sustainability of package delivery and its worldwide CO2 contribution to climate change. Our solution is but one path to addressing the great challenge of global CO2 reduction, but we believe it is a technically feasible, effective and advantageous business approach by enabling the safe drone delivery of packages using renewable energy.
2 Comments
Please login to post a comment.
keith woollvin
This is a really interesting concept, and it looks like the FPGA was the perfect choice for the application. If I read this correctly, the project is capable of acquiring hazard information, but is it capable of affecting a route change? Its impressive what you have achieved so far.