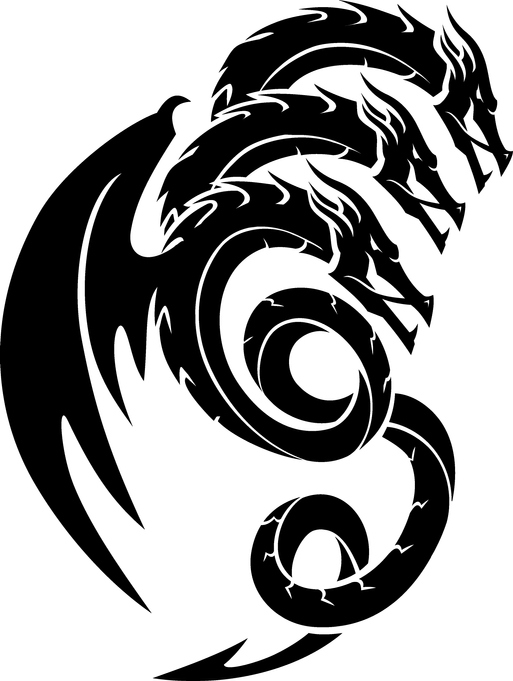
Designe special version of RISC-V MCU with three cores and special supervisor logic. The cores will work in parallel and independent with same as programm. The special logic part controls result of calculations and outputs for detect broken core/result. The best result is do working device with broken some parts by any reason and restore working all cores if possible.
Project Proposal
1. High-level project introduction and performance expectation
Problem:
Nuclear particles from space or from a nuclear source may change working state of electronic device. There are 3 basic cases:
- - The changing RAM bit state, logic state or flip-flop state to another 0 -> 1 or 1 -> 0. It is non-fatal problem.
- - The thyristor effect or short cicrcuit in-out. It is potential fatal problem.
- - Damage of the semiconductor structure. It is fatal problem.
Some technique are used to protect electronic devices which work in places with the risk of radiation. It is shielding, modular reservation, internal reservation, special semiconductor hard-rad solutions.
This research project tries to find cheap and simple way for solve this problem.
Solution:
As core we will use open RISC-V core. This core is opened for any modifications and task optimization, without any fees or additional payments.
Simple SoC with RISC-V core will have:
- - SPI bus for execute a program from an external SPI Flash
- - SPI bus for communication with external devices like ADC
- - UART for communication with a control center
- - Some timers
This SoC is one head of our dragon. For fail-safe working we will use internal triple reservation of running SoCs it will be three headed dragon. All input signals will go to each SoC in parallel from IO matrix. All output signals from SoCs will go to IO matrix majority logic for provide output signals.
Special supervisor control logic will check every tick states of SoCs. If there are any problems found with any SoC it will stoppen and show what happened and which SoC with by simple logic output interface.
Internal dual-core ARM MCU of DE10-Nano kit will be a control center. One of its function is to read state of three headed dragon, to send it to Azure cloud, receive command from cloud and to do something else.
2. Block Diagram
The project will be based on simple RISC-V SoC design.
Execute a program from an external flash and has few peripherials for commutication with external devices.
But for robust and fail-safe operation we will used three same as SoCs in one chip. With special supervisor circuit for control.
3. Expected sustainability results, projected resource savings
1. Do working simple RISC-V SoC for execute a program from an external flash memory.
2. Design simple programm for capture data from an external ADC, calculate real value and send result over UART.
3. Design simple supervisor controller for check state of heads.
4. Do working all three SoC with same as program.
5. Do working simple program with full functionality of heads and with fail one of them.
0 Comments
Please login to post a comment.