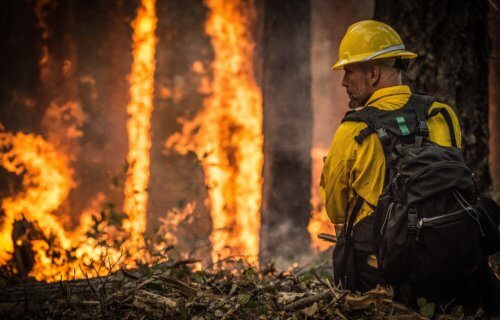
Recently, forest fires are on the agenda of a lot of countries. A significant portion of forests in tropical zones is facing a risk of burning down. To help to respond quickly to fires, a cloud-based IoT solution could be used. Current fires will be sensed with a camera and CO2 sensor which will use either NDIR or electrochemical technology. System alerts authorized personnel about risky and emergencies over Cloud. Additionally, notable environmental factors are oxygen, combustible gases, humidity, and temperature, so sensors for these factors will be used to collect environmental data to predict potential forest fires beforehand. This collected data will also be available in the Cloud. System alerts authorized personnel about risky and emergencies over Cloud.
Cover image by skeeze on Pixabay
Demo Video
Project Proposal
1. High-level project introduction and performance expectation
Forest Environment Analysis to Detect and Predict Forest Fires
Forest fire is one of the main environmental issues that deeply affect the ecology. On 7 February 2009, bushfires in southeast Australia burned 450,000 hectares and resulted in 173 deaths.[2] Therewithal, recently, wildfires are on the agenda of a lot of countries. As it is known, the fires that broke out in the recent past in Turkey were difficult to bring under control and many forest areas and lives were lost. A significant portion of forests in tropical zones is facing a risk of burning down. The purpose of this project is to create a fire prediction system as well as a detection system by combining the data taken with oxygen, humidity, temperature, flammable gas, CO2 sensors, and a camera. A cloud-based IoT solution can be used to help detect and respond to fires quickly, even predicting and preempting them.
Current fires will be sensed with IR and CO2 sensors which will use electrochemical and photoelectric sensor technology. System alerts authorized personnel about risks and emergencies over Cloud. Additionally, Notable environmental factors are oxygen, combustible gases, humidity, and temperature, so sensors for these factors will be used to collect environmental data to predict potential forest fires. This collected data will also be available in the cloud.
In a survey, it was determined that if the fire had been detected at the time of the fire, 80% of the losses from the fire would have been kept away[1]. The key reasons for the need for this system and the design idea are to protect the habitats of wild animals and prevent the destruction of forests and loss of life and property.
Related Studies:
In recent years, a large number of responses have been viewed and executed for out-of-control fire detection. In a Fire Detection System, Video Surveillance System is one of the fundamental methods used to identify forest fires. It is divided into four classifications; Infrared (IR) Thermal Imaging Cameras, Video Cameras, IR Spectrometer, and Light Detection and Ranging (LIDAR) [1]. However, these technologies were limited by a high false alarm rate caused by climatic circumstances such as fog, shadows, clean particles, etc. Another method is to use a satellite system to determine the location of the conflagration. The satellite(s) and the base station, which collects the data sent by satellite(s) and performs the dissection calculation, are the two main components of the framework[1]. The mists and clouds, on the other hand, have a significant effect on the frame, blocking the image taken.
References
[1] M. Trinath Basu, Ragipati Karthik, J. Mahitha, V. Lokesh, Reddy International Journal of Engineering & Technology, 7 (2.7) (2018) 124-126 [Online] Available: 10.14419/ijet.v7i2.7.10277
[2] M. G. Cruz, A. L. Sullivan, J. S. Gould, N. C. Sims, A. J. Bannister, J. J. Hollis, and R. J. Hurley, “Anatomy of a catastrophic wildfire: The Black Saturday Kilmore East Fire in Victoria, Australia,” Forest Ecology and Management, 31-Aug-2012. [Online] Available: https://doi.org/10.1016/j.foreco.2012.02.035
2. Block Diagram
3. Expected sustainability results, projected resource savings
In a survey, it was determined that if the fire had been detected at the time of the fire, 80% of the losses from the fire would have been kept away [1]. The purpose of the “Forest Environment Analysis to Detect and Predict Forest Fires Project” is to build a fire detection and also prediction system with the help of gas sensors such as oxygen, combustible gases, humidity, temperature, CO2, and IR sensors to prevent wildfires. Instead of only a detection system, a prediction system is envisioned. For that reason, a prediction before any potential fire risk will decrease the risk of wildfires for all forested lands, and also a prediction helps to make direct provision for wildfires.
[1] M. Trinath Basu, Ragipati Karthik, J. Mahitha, V. Lokesh, Reddy International Journal of Engineering & Technology, 7 (2.7) (2018) 124-126 [Online] Available: 10.14419/ijet.v7i2.7.10277
4. Design Introduction
The purpose of this project is to create a fire prediction system as well as a detection system by combining the data taken with oxygen, humidity, temperature, flammable gas, and CO2 sensors. A cloud-based IoT solution can be used to help detect and respond to fires quickly, even predicting and preempting them. The targeted users of this project are the government, nature conservation foundations, or private woodlands.
5. Functional description and implementation
The device will collect information from sensors to detect and predict potential forest fires. The FPGA processes oxygen, humidity, temperature, and volatile organic compound sensors to predict a potential forest fire. In addition that, FPGA will detect current forest fire by using CO-AX(CN0357) and infrared flame sensors. The built system performs threshold detection. In addition, the FPGA can implement threshold detection, slope detection, and seasonal calibration. Slope detection can be used for detecting instantaneous changes which can be caused by unexpected situations such as wildfire. Additionally, Threshold Detection perceives environmental situations prone to wildfire. It is aimed to deploy this system in every forest but habitats of rare species and known dangerous areas are prioritized. Locations of the devices are either saved to the cloud while deployment, or triangulated on-field if both hardware and environmental conditions are suitable. The system might be powered by rechargeable batteries charged by solar panels. In dense forests, devices on the ground might not get enough sunlight, so to make sure they get sunlight nodes placed manually which means saving locations manually is more applicable. Contrarily, in sparse forest nodes can be spread from a plane, and locations triangulated to decrease deployment labor. To decrease the wildfire risk from the combustion of the rechargeable battery, a fireproof case should be used. Another benefit of using the fireproof case protects internal electronics from wildfire to potentially reuse.
As it is known, the main purpose of the project is to detect and predict forest fires, and to prevent large and irreversible forest fires, which are quite difficult. In the first step of the solution proposal, the system to be created for this problem was provided by reconfiguring the Nios II system files and the RFS module in the Module5_Sample_HW file on the Terasic GitHub page. In addition to the example Nios II system in the file, the 2 ADC- number of the ADC can be increased up to 8-,1 Interval Timer, SPI, and inputs and outputs have been added. The "nios_qsys.qsys" file, has been reconfigured for the FPGA configuration. Also, the Nios code -from Eclipse- has been changed to suit the system. Then, Pinout connections were defined with the help of the "de10nano user manual" and "de10nanomaster.qsf". An Azure deployment had to be created to see data from sensors on the cloud. For this reason, device provisioning was needed on Azure. Next, an IoT Edge Module had to be created for the solution. For this, we needed to configure a deployment manifest. In short, we modified Terasic and Azure Reference Applications as required. Right now it works on IoT Edge configuration. We are unsure that its ready for central deployment.
We had to remove the camera and some sensors from our solution due to the economic reasons that emerged in our solution and some problems caused by the pandemic in Turkey. We had to build the system with the sensors we already had. Our solution is an IR sensor and a gas sensor added. Also, the solution can be solved by adding other sensors to the ADC and reconfiguring the name. We don't have a combustible gas sensor and oxygen sensor, but we compensated for missing sensors with ADC messages to the cloud which can be processed later.
Source Codes can be found on Github. (here)
6. Performance metrics, performance to expectation
Field programmable gate arrays (FPGAs) applications are increasingly being implemented particularly because for real-time embedded applications, in which latency and power consumption are critical factors. Emergencies, either potential fire or current fire, are found by processing sensors data with the FPGA. To describe another benefit of FPGA is to execute FPGAs applications in the cloud using software tools, hardware kits, and remote FPGA platforms. FPGA is integrated into the cloud to benefit from its resources to support cloud infrastructures. Taking advantage of the cloud's "infinite" capabilities, numerous application designers can efficiently use FPGA resources. To evaluate FPGA benefits for this project, FPGA can perform efficiently with sensors such as Oxygen sensor, Humidity sensor, Temperature sensor, Volatile Organic Compound Sensor, and CO-AX sensor as it is shown in the block diagram. The most important feature of FPGA while performing these processes is performing with minimum data corruption.
7. Sustainability results, resource savings achieved
8. Conclusion
As a result, a solution was designed in this project to install a fire detection system as well as a fire prediction system and intervene to prevent forest fires. In this way, a system is created that will minimize both the loss of life of wild animals and humans and the loss of forestland.
1 Comments
Please login to post a comment.
EM021
https://github.com/obsunal/InnovateFPGA-EM021-Wildfire
Link to Source Codes