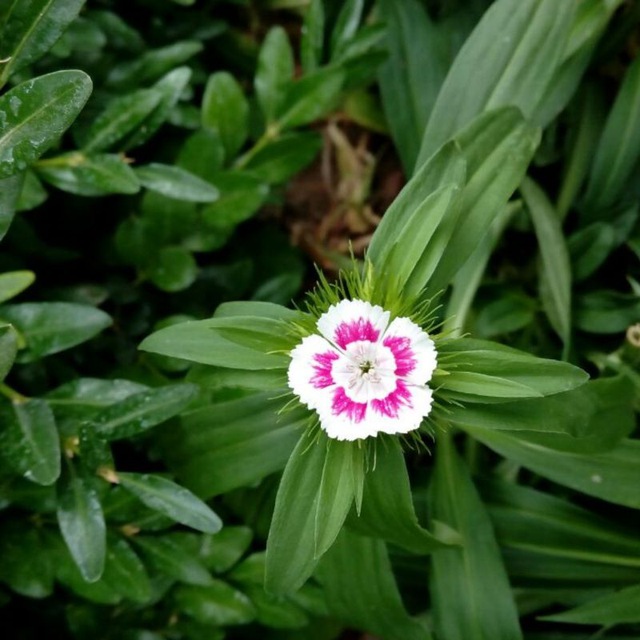
It is about how we have a good garden , how we have healthy food, and how we do them in smart manner .
Project Proposal
1. High-level project introduction and performance expectation
2. Block Diagram
3. Expected sustainability results, projected resource savings
4. Design Introduction
5. Functional description and implementation
6. Performance metrics, performance to expectation
7. Sustainability results, resource savings achieved
8. Conclusion
0 Comments
Please login to post a comment.