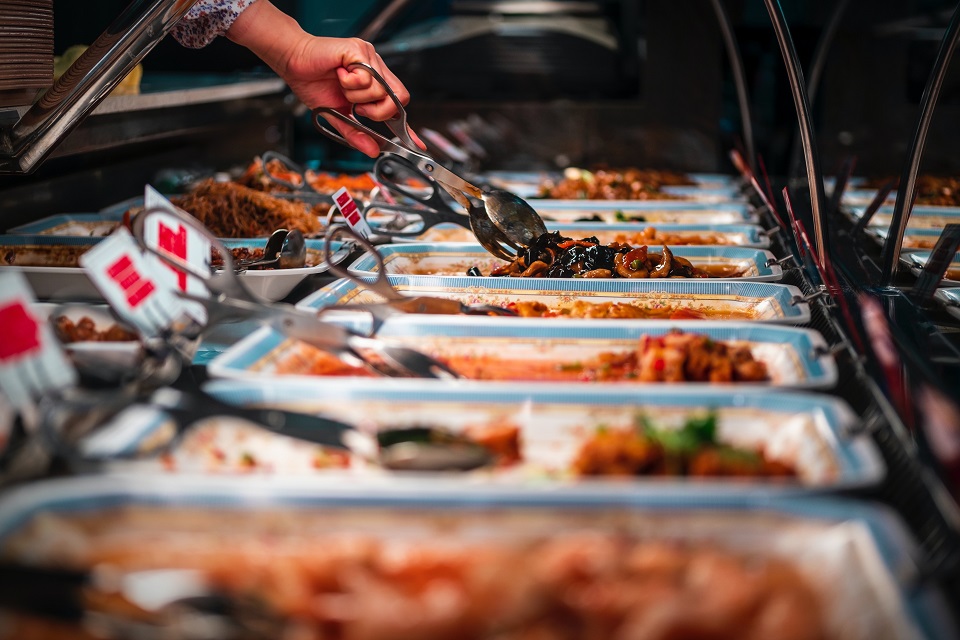
Food Saver aims to reduce food waste in resorts and hotels by preventing overproduction and improving storage and service conditions.
Demo Video
Project Proposal
1. High-level project introduction and performance expectation
Hotels and resorts give exaggerated services to satisfy the customers and overlook enormous waste, especially for food. Food Saver aims to reduce food waste in resorts and hotels by preventing overproduction and improving storage and service conditions in excess of 10 billion US dollars annually.
Five significant areas contribute to food waste:
· Storage Losses: The food wasted due to improper and unhygienic storage conditions.
· Preparation Losses: The food mainly consists of seeds, peels, etc., from fruits and vegetables.
· Serving Losses: The food left on serving dishes, in canteens and bowls.
· Overprepareds: The food that is never served.
· Leftovers: The food that the customer leaves on the plate.
Food Saver focuses on the following three points:
- Overprepareds
Overprepareds occur when the production of the food is higher than its consumption. Estimating the required amount of food supply is a crucial part of the problem. To overcome this problem, Food Saver aims to utilize the basic correlation between the number of people and the food consumed. Food Saver uses the motion sensors mounted on the doors to count those entering and leaving every meal. Then it sends the acquired statistics to the cloud. Then, the cloud estimates the required food. Over time, accumulated data will be helpful to train an AI-based algorithm to predict the required amount of food even before months.
- Serving Losses
The served food must preserve its temperature and moisture for customers' utmost satisfaction, while the food waiting to be served requires the opposite to prevent the growth of mold and other bacteria. Also, the food that is served must be replaced regularly to meet food health standards. So, the problem is the food has to be prepared in large quantities due to timing constraints, but it also should not wait a long time in serving conditions. Food Saver aims to solve this problem by utilizing a serving mechanism that consists of temperature-controlled divisions. Conditions of these divisions are controlled such that the short-term serving foods are contained in optimal temperature divisions. In contrast, longer-term serving foods are held in lower temperature divisions. Food Saver detects when the top division is emptied and ascends the lower division for the serving by using weight sensors. Hence, Food Saver facilitates the food serving process while keeping a higher shelf life.
- Storage Losses
Improper storage conditions such as high temperature and moisture lead to mold, and mold releases CO2 (Hesseltine, 1976). Food Saver aims to prevent storage losses by monitoring the temperature, moisture, and CO2 conditions of the food prepared in large quantities. AI algorithm will check the dependency of these parameters in real-time to alert and activate cooling and ventilation systems that will be integrated with UPS power systems (Dagnas & Membre, 2013).
Food Saver sends all the acquired data to the cloud. Hence, it can be a part of a more intelligent, complex, and interconnected network by connecting multiple nodes.
2. Block Diagram
3. Expected sustainability results, projected resource savings
The USA wastes 63 million tons of food worth 218 billion dollars in a year (Briggs, 2016). In this design, our focus is the waste in consumer-serving businesses like hotels and restaurants, which sums up to 40 percent of whole food waste (Himelstein, 2017). Our project will significantly reduce food waste and eliminate unnecessary transportation, workforce, storage area, and decrease carbon dioxide emission. Total value proposition of our solution is well above 8 billion USD and saver of green pastures and part of ecosystem preservation act called Green Deal compatible.
REFERENCES:
- Dagnas, S., & Membre, J.-M. (2013). Predicting and Preventing Mold Spoilage of Food Products. In Journal of Food Protection (Vol. 76, Issue 3, pp. 538–551). International Association for Food Protection. https://doi.org/10.4315/0362-028x.jfp-12-349
- FSEC Energy Research Center. (2020, August 12). Mold Growth. Retrieved November 4, 2021, from https://energyresearch.ucf.edu/consumer/buildings/building-science-basics/mold-growth/
- H. Briggs, J.D. Lindeberg, A. Rein, B. Chorn and K. Tanger. (2016, June 1). The ReFED Roadmap To Reducing Food Waste. Refed. Retrieved October 30, 2021, from https://refed.com/articles/the-refed-roadmap-to-reducing-food-waste/
- Himelstein, L. (2017, October 4). Hotel Buffets, a Culprit of Food Waste, Get Downsized. The New York Times. https://www.nytimes.com/2017/09/08/dining/hotel-buffet-food-waste.html
- Hesseltine, C. W. (1976). Conditions Leading to Mycotoxin Contamination of Foods and Feeds. In Advances in Chemistry (pp. 1–22). AMERICAN CHEMICAL SOCIETY. https://doi.org/10.1021/ba-1976-0149.ch001
- Okumus, B. (2019). How do hotels manage food waste? evidence from hotels in Orlando, Florida. In Journal of Hospitality Marketing & Management (Vol. 29, Issue 3, pp. 291–309). Informa UK Limited. https://doi.org/10.1080/19368623.2019.1618775
- Reynolds, C., Goucher, L., Quested, T., Bromley, S., Gillick, S., Wells, V. K., Evans, D., Koh, L., Carlsson Kanyama, A., Katzeff, C., Svenfelt, Å., & Jackson, P. (2019). Review: Consumption-stage food waste reduction interventions – What works and how to design better interventions. In Food Policy (Vol. 83, pp. 7–27). Elsevier BV. https://doi.org/10.1016/j.foodpol.2019.01.009
- Youngs, A., Nobis, G., & Town, P. (1983). Food waste from hotels and restaurants in the U.K. In Waste Management & Research (Vol. 1, Issue 4, pp. 295–308). Elsevier BV. https://doi.org/10.1016/0734-242x(83)90034-4
4. Design Introduction
5. Functional description and implementation
forever:read framedetect objects every couple of framestrack objectsdisplay
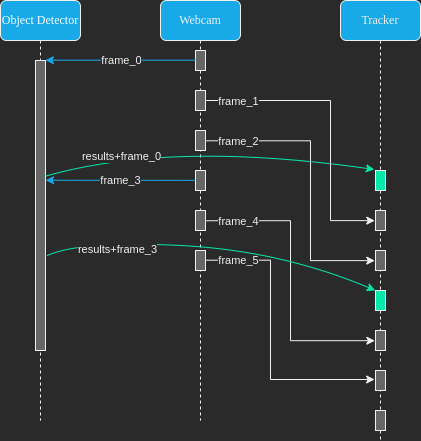
- The webcam thread produces an unknown number of frames between each object detection. The size of the frame packet depends on object detection speed. The longer the detection takes, the more frames are produced.
- The object detection thread must read the most recent frame.
- All threads have to synchronize at the end of object detection.
- Threads must not eat away CPU resources when they are waiting for synchronization.
- There can't be a large number of duplicate frames.
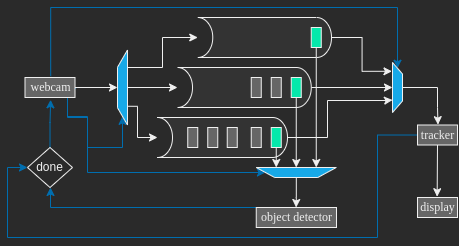
6. Performance metrics, performance to expectation
7. Sustainability results, resource savings achieved
Our design's detection capabilities are already behind statistical predictions. However, even if it was operating with a %100 detection and tracking rate resource-saving would still be minimal. Overprepareds are only a small portion of the wasted food. In addition to that predicting number of people is not enough to accurately estimate the amount of food that will be consumed. Which further degrades the significance of the system. Still, collecting data can and probably will be useful for future studies aiming to tackle the wasted food problem.
8. Conclusion
This study has several limitations. First, even though the usage of the gas sensor array is eliminated by deeming it unfeasible, such a system can be implemented by using high-quality sensors, particle filtering and sensor ventilation, and a large dataset. Second, object detection and tracking performance are measured with custom recordings and can not be used to ensure reliable operation in all conditions.
In summary, this paper has investigated different methods to prevent food waste in hotels and resorts. And concluded that food waste prevention requires cheap and easy to enforce solutions. Even reducing one source of food waste requires an overhaul of the current food serving norms. Hence, such methods are not only economically unviable but also unreliable.
0 Comments
Please login to post a comment.