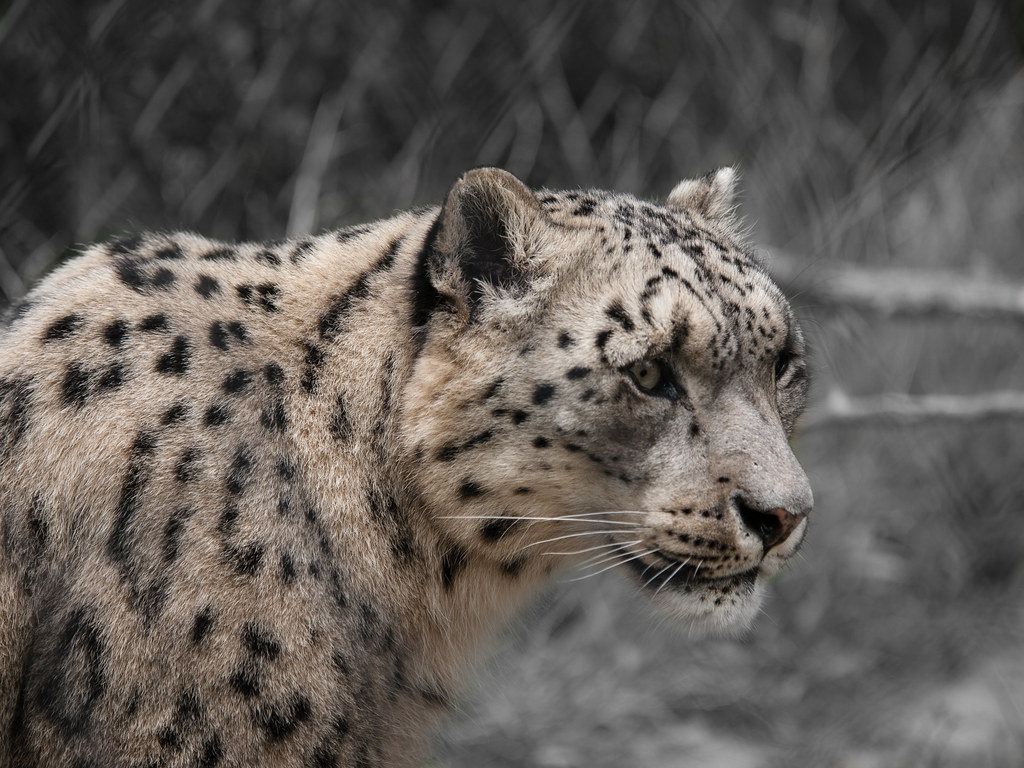
The goal of this project is to develop an AIoT based platform to detect and track snow leopards in Sanjiangyuan region, China.
Project Proposal
1. High-level project introduction and performance expectation
Currently, camera traps are used to identify species and individual animals in the wilderness. This involves huge manual effort, both in gathering the collected data from the cameras as well as analyzing the data afterwards. With our platform we combine the strengths of FPGAs and cloud based AI solutions to drastically reduce the effort in data collection as well as data analysis.
Our platform consists of three main components. At first, there are intelligent monitoring stations, which can be placed in a region of choice. All stations will report preprocessed data to an Azure cloud instance, which is the second component of our platform. There will be an automated, extensive data analysis including anomaly detection within the Azure cloud from data of all monitoring stations. The third component of our platform is a user-friendly dashboard, which supports scientists in analyzing the habits of snow leopards.
The video data from the camera will be preprocessed and uploaded to the cloud. Along with the preprocessed data from the camera, the identification of the station will be send to the azure instance. This allows the geographical mapping of gathered data.
The uploaded data will be stored in a data store. An AI model is trained and used to classify the individual snow leopards (multi-class classification). As training and test data the available 5,000,000 photos and videos of 100 individual snow leopards are used. The output of the model is stored along with the gathered preprocessed data.
Our first, major milestone is the realization of a technology demonstrator to show the feasibility of our platform for snow leopards tracking. The demonstrator consists of a single monitoring station, an Azure cloud instance and a user-friendly dashboard. The Azure cloud instance and the dashboard will already support several monitoring stations for the first milestone. After the first milestone has been achieved, the system can be easily scaled by adding more monitoring stations to the platform.
2. Block Diagram
The DE10-Nano board from Terasic is the core component of our monitoring station. Additionally, several external modules are connected to the DE10-Nano board:
- An 8MP MIPI camera is considered for capturing video frames. Therefor, the D8M-GPIO module from Terasic will be applied.
- Preprocessed data will be transferred to the Microsoft Azure cloud via the RFS kit.
- Environmental conditions such as temperature and humidity during snow leopard tracking will be fetched from sensors of the RFS kit.
- An external USB GPS sensor will be applied to determine the exact position of the monitoring station.
In order to provide maximum flexibility in the positioning of the monitoring station, the complete station is battery powered. The batteries are recharged by an off-grid solar system. However, the off-grid solar system is out of scope of this work.
Local data processing is done within the Intel Cyclone V SoC-FPGA. Whereby HW/SW-Codesign methods will be applied to achieve an optimum system design. A custom Linux OS will be developed by means of Buildroot, to achieve a minimum, tailor-fit operating system. An application within Linux user space will control the complete monitoring station. The interface to the FPGA portion will be handled by loadable kernel modules.
3. Expected sustainability results, projected resource savings
Our platform shall reduce the manual efforts for snow leopards tracking significantly. In order to achieve this, the monitoring stations of our system have to be set up only once. Afterwards, there is no regular on-site service required. This is a big benefit compared to the current solution. The current solutions relies on manual data gathering from camera traps.
Furthermore, there is a also a huge advantage in the update cycle of the data. We want to achieve a daily update of the dashboard, which displays all relevant data from all monitoring stations. Currently, the data are only updated after several months, because the data has to be gathered manually.
References:
"Snow Leopard" by wwarby is licensed under CC BY 2.0
https://www.innovatefpga.com/portal/news/sgp-1.html
4. Design Introduction
5. Functional description and implementation
6. Performance metrics, performance to expectation
7. Sustainability results, resource savings achieved
8. Conclusion
0 Comments
Please login to post a comment.