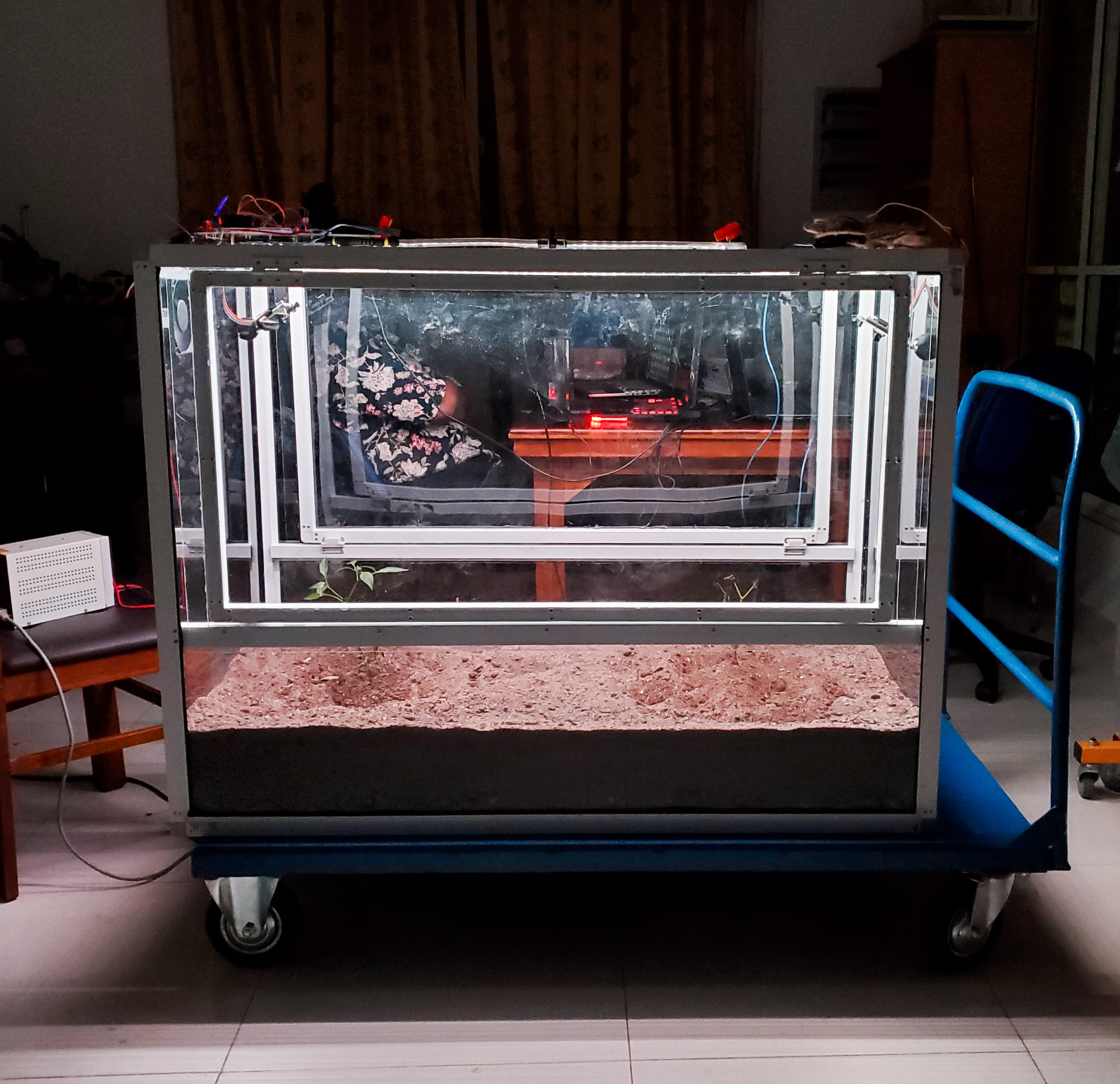
Our project aims to provide a smart, user-friendly, domestic mini-greenhouse management system to enable users to grow and efficiently monitor and tend to plants within their own homes. This encourages people to grow their own food plants by removing the difficulties related to gardening in the busy lifestyle.
Demo Video
Project Proposal
1. High-level project introduction and performance expectation
Purpose of Design
With the climate changes and the increase of population, the demand for food supply has seen a rapid increase throughout the past years. Therefore, the attention has been given to developing smart technologies like precision agriculture to improve the quality and yield in large scale agricultural farms. However, as the demand increases, it has been challenging to match the supply with the demand and to maintain the ever-expanding supply chain. Also, with the world facing a severe pandemic situation, many countries in the world have faced a crisis of food shortage and breakdown in the supply chain.
Our solution aims to provide a smart and automatic mini-greenhouse management system for food plants targeting the local urban community. There are conventional systems without automatic control already available in the market (in the figure above). However, they have not been commonly adopted due to the difficulty in continuously maintaining them in the busy lifestyle. Our goal is to bridge this gap and use electronic control to monitor and tend to the plants. This will encourage more users to adapt to this concept of growing their own food. Also, this will help to supply fresh, organic food from their garden which the users can safely consume. This will improve their quality of life.
Application Scope
The basic functionality and the monitoring aspects of our system are as follows.
The data from the sensors will be sent to the FPGA to process and determine the requirement of irrigation, fertilization, nutrients, ventilation and light at the edge.
Camera footage will be used to allow users to remotely observe their mini-greenhouse, track plant growth, predict yield and identify abnormal behavior such as over-growth.
This data will also be sent to a cloud database hosted on Microsoft Azure, where they will be efficiently stored and processed to monitor the performance of the mini-greenhouse in the long run.
Using the flexibility of the FPGA and the abundance of sensors available, monitoring aspects can be expanded in the future using system updates.Also, a centralized database could be created in the cloud with data from multiple gardens in a local community to better share and manage their yields.
Targeted Users
We mainly focus on developing a product for the average domestic urban household. A single FPGA can cater to several mini-greenhouses within its vicinity. Therefore, small-scale farms can also benefit from this technology.
Why do we use an Intel FPGA?
For the control system, we specially consider using an Intel CycloneV FPGA because of the following reasons.
-
Low power consumption
-
High computational power for a lower cost
-
Reconfigurability
- Combination of Hard Processor System (HPS) and FPGA
To test our implementation, we will use the DE-10 Nano development kit from Terasic.
We will need to perform amplifications and analog to digital conversion on sensor data in high precision and speed. Also, we expect to monitor environmental factors such as temperature, humidity, light intensity, etc. For these purposes, we plan to use sensors and evaluation kits such as CN0398 from Analog devices.
2. Block Diagram
The design we propose is a smart automatic management system for mini-greenhouses that could be used in urban households, apartments and small-scale farms to grow food plants. The environment is controlled within a confined space with appropritely controlled conditions.
The system block diagram is illustrated in the following figure. It presents the environmental conditions we monitor, how the sensor data is handled and control variables of our system.
The following diagram illustrated how the CycloneV SoC is being utilized to handle and process data.
3. Expected sustainability results, projected resource savings
Our project relates to two sustainable development goals (SDG);
Goal 2: Zero Hunger
Food security is achieved by allowing users to determine their own chemical levels and have fresh organic diets with improved nutrition. Through our project, the urban community will also start contributing to promote sustainable agriculture.
Goal 12: Responsible Consumption and Production
The emission of greenhouse gases can be reduced and eco-friendly food production methods will be used. Also, by sharing the excess produce within the local community, the wastage of food can be minimized.
Expected Sustainability Results
Implementing smart and efficient management of mini-greenhouse
Higher food productivity
Decrease excessive use of chemicals
Encourage and increase organic food consumption
Simplify the supply chain
Increase agricultural exports and boost the economy
Resource Savings
Reduced wastage of agricultural products
Efficient use of water
Reduced use of natural resources to maintain a complex supply chain
- With adapting to our system, more people will be encouraged to maintain their own gardens. This will enable them to;
Get fresh produce right from their home mini-greenhouses which will give health benefits to the consumer.
Improve their mental and physical well-being by enjoying a fruitful garden right at their home and the ability to save time otherwise spent to manage a garden
-
Through our system, the user can choose the level and type of fertilizers and pesticides used in their garden which will assure them about the quality of food and reduce the risk of consuming harmful chemicals.
-
By adopting our technology on a larger scale, the excessive use of chemicals and emission of greenhouse gases can be reduced by efficiently managing those parametrics.
-
The data obtained from multiple gardens in a local community can create a local community market where the data stored in the cloud are centralized, processed and provided to users to share their extra yields within the market. This will greatly reduce the wastage and provide the users a means of income as well.
-
Implementing these kinds of local markets can reduce the supply chain complexity thus reducing the resources like fuel which is consumed to maintain a vastly spread supply chain. Also, as an added benefit, the warehouse wastage can be greatly reduced.
-
In the long term, the general supply chain can be interrupted because the local communities would be able to sustain on their own. Therefore, the government can increase its exports of agricultural products yielded from large scale farms to develop the economy.
4. Design Introduction
Agriculture is the way of cultivating soil and growing crops to provide food, the most basic requirement for human survival. Over one third of the world population is employed in agriculture. The following two sustainable development goals (SDG) by the UN focus on promoting and improving the agricultural field.
Goal 2 (SGD2): Zero Hunger
SDG Zero hunger is focused on promoting sustainable agriculture, supporting small-scale farmers and equal access to land, technology and markets. With the global population projected to touch 9.6 billion by the year 2050, a growth of 70% in global food production is needed to meet the increasing food demand. [1] It also requires international cooperation to ensure investment in infrastructure and technology to improve agricultural productivity. This goal aims to end hunger and malnutrition of the ever-growing population in the world.
Goal 12 (SGD12): Responsible Consumption and Production
Through this SDG, the United Nations Development Programme has identified that agriculture is the biggest user of water worldwide, and irrigation now claims close to 70 percent of all freshwater for human use. The efficient management of our shared natural resources, and the way we dispose of toxic waste and pollutants, are important targets to achieve this goal.
[2]
However, most of the world still makes use of traditional farming methods without adopting the cutting edge technology available to achieve these targets. This makes the process of agriculture very inefficient. Our country, Sri Lanka, also heavily depends on these traditional farming techniques and we are currently experiencing its consequences with the ongoing food shortage. However, a World Bank report in 2019 argues that developing countries need to “dramatically” increase agricultural innovation and the use of technology by farmers in order to eliminate poverty, meet the rising demand for food and adapt to climate change. [3] At present South Korea, China, North America are investing trillions of money for development in the agriculture sector and implementing more advanced technologies. [4]
We introduce a much more efficient way of farming available to everyone by making the best use of technology. Through this solution, we will be able to make agriculture a part of everybody’s life. Specifically, it provides an exciting and fun way of farming which is adoptable by an average person without much hassle. Therefore, our solution can be adopted by a vast community of domestic users to grow a considerable portion of their food within their homes with the reassurance of food safety and reliability. This will pave the way to end hunger and malnutrition in SDG 2 by increasing the sustainable productivity of food. Furthermore, being smart and efficient, our solution will be able to save much of the natural resources wasted in the field of agriculture and reduce the usage of pesticides to achieve SDG 12.
To achieve these objectives, our solution has the following characteristics.
Affordable
Modular and Scalable
Efficient
Integrated with the latest technology
An IoT solution
Easy to use
Fun to use
We introduce an automated mini greenhouse system which harnesses the power of DE-10 Nano and internet of things to achieve the above objective. This enables the users to grow and efficiently monitor plants at the reach of their hands.
Our system makes use of virtues from edge computing as well as cloud computing to obtain efficiency and performance. The combination is used to overcome disadvantages in both technologies.
The distinguishing characteristics of Edge Computing from Cloud are dense geographical distribution, mobility support, location awareness, proximity, low latency, context-awareness, and heterogeneity. [5] As the data is mainly processed at the edge using the DE-10 Nano, the latency is reduced in reacting to sudden changes within the greenhouse. Also, the dependence on the internet connectivity is minimized by using edge processing and using Azure IoT Edge which can store data for a period of time and transfer data when the internet connection becomes available.
The sensor and image data are transferred to the cloud after processing for long term monitoring and system improvements. The amount of memory required to store large amounts of data in the long run is avoided by storing them in the cloud and superior tools like Machine learning and database management provided by Microsoft Azure platform are efficiently used to process and make necessary decisions to improve the system based on these data.
Purpose of Design
Affordability
Our mini greenhouse system is designed in a cost effective way for it to be affordable and reachable to every average user. This includes having low maintenance costs and low power consumption. By making our system adaptable by many users, the overall improvement of efficiency could be maximized.
Modularity and Scalability
This characteristic of our design enables the user to increase their engagement in agriculture by expanding and combining multiple units of such greenhouses. This can make our system suitable for large-scale agriculture that involves indoor or vertical farming.
Efficient
Our mini greenhouse system is designed to optimally use the resources to obtain the maximum growth and produce out of plants within a minimal time duration. This saves much of resources like water and nutrients being wasted and prevents their insufficient or excess usage from negatively impacting the plants.
Adaptive to latest technology
We design our mini greenhouse system to be upgradable and adaptable to the latest technology. The system will be maintained up to date to make the best use out of information made available through research and advancements.
IoT solution
We integrate the internet of things into our system to store and process the plant data in the cloud to monitor them over time. Additionally, this will enable our system to react intelligently and instantly to degrading conditions in the plants by analyzing the stored data.
Easy and fun to use
We make our system to be used by an average person without much experience in agriculture. And it is designed to be attractive and fun to use. This is with the purpose of increasing the adaptability and engagement of people in agriculture.
Basic Functionality
Our mini-greenhouse has the following basic functionality.
Automatic irrigation
Controlling light for the plants for optimum growth and yield
Ventilation and temperature regulation
Tracking the plant quality and growth with time
Warning the user of sudden changes in the conditions within the greenhouse
Gathering of data and maintaining a database In Microsoft Azure for monitoring and predictions
Application Scope
Operating as an automated greenhouse where the plants can grow under an optimal environment to achieve highest yield.
Expanded up to large scale indoor and vertical farming by combining multiple units.
The gathered data from the greenhouse will be stored in Microsoft Azure. These can be used to study the behavior of plants under certain environmental conditions.
Plant based research which may include tracking the growth and production using the data recorded under controlled environments.
For exhibition and individual relaxation purposes
Targeted Users
Traditional farmers
Our system could be introduced to traditional farmers as a new and more efficient way of maintaining cultivation.
Home owners
Homeowners can adopt our mini greenhouse system for indoor farming to grow a portion of their own food without the hassle of tending to it.
Researchers
Researchers can make use of this mini greenhouse system to study the behavior of plants over time under a controlled environment.
Why do we use an Intel FPGA?
The system will be controlled by a centralized controller. We identified the following features as the capabilities our controller should possess to manage the required functionality as an IoT solution.
I/O capability
Computational power
Networking capabilities
A graphical user interface
An architecture that is upgradable
Affordable
Compact with low power consumption
Considering the above features, we identified an FPGA to be the most efficient controller to implement our system. Specifically, the following capabilities of Intel SoC FPGAs were utilized to obtain the above functionality.
I/O Expansion
We design our system to be modular and scalable such that several modules of the greenhouses could be connected to the central controller. Therefore, we can make use of the extended number of I/O pins available on the FPGA which are configurable as required.
Computational Power
Our control algorithm consists of both sequential and parallel computations. The sequential code could be implemented in the HPS portion of the SoC while the FPGA portion is utilized to accelerate and parallelize computations like matrix multiplication in the implementation of the neural network. This immensely improves the performance of our system.
Network Capabilities
As an IoT solution, our system greatly benefits from the networking capabilities of the Intel FPGAs. This enables our system to connect to the internet to communicate with the cloud and to be remotely reconfigured via updates. Additionally, the RFS board designed for Intel FPGA development boards makes the process of networking much more efficient and convenient.
A GUI
The ability to provide the users with a graphical user interface is very important for our mini greenhouse system for the user to easily monitor and control the conditions of the plants. The HPS portion of Intel SoC provides the display and control capabilities for this. A lightweight Linux operating system or a custom designed bare-metal GUI could be implemented in the HPS to allow the user to easily manage the greenhouse without much technical knowledge.
Reconfigurability
The reconfigurability of the FPGAs enable our architecture to be upgradable after the initial implementation. The data stored in the cloud is processed in the long term to improve the design architecture to achieve more optimal yield. These upgrades can be done remotely through the internet using container based applications. This makes our system up to date with the latest technology and newest findings through research.
Affordability
The cost of designing and maintaining a complex control system on an FPGA is less compared to other processing devices like microcontrollers. Microcontrollers require additional memory and external modules to achieve the same computational and other capabilities required by our system, therefore increasing the overall cost and complexity of the implementation.
Size
The smaller size and the low power consumption of FPGAs lead to a compact and efficient solution.
To support our idea, [6] shows how their Cyclone IV FPGA based computer system for greenhouse control has proven superiority over existing systems by reducing the cost while at the same time increasing versatility.
A study [10] done on current usage of technology of smart farming mentions that most current systems are based on microcontrollers and cloud computing. Even though FPGAs are currently not popular in the agricultural field, its computational power can be harnessed to perform powerful edge computing while being an IoT solution.
To test our implementation, we use the DE-10 Nano with Cyclone V SoC development kit from Terasic.
5. Functional description and implementation
To comply with the above objectives, we have come up with the following design of a mini-greenhouse system. A single green machine is equipped with a set of cost effective sensors and actuators to monitor and control the condition within the greenhouse. All of these sensors and actuators are connected to a microcontroller within a green machine. The complete control system is implemented on a Cyclone V SoC, to which multiple such green machines can be connected. The microcontrollers communicate with the central controller via Bluetooth. The FPGA central controller determines the necessary requirements of water, ventilation and light based on the sensor and image data from each greenhouse and sends control signals for the actuators via the same Bluetooth link. Furthermore, the received data of each green machine is sent to the Microsoft Azure database for further analysis.
Now, the control flow of a single green machine is explained below.
Sensor Integration
According to the research and experiments in [6] [8] [11-13], we identified the following sensory measurements to be vital in monitoring the conditions within a greenhouse.
Environmental temperature
Environmental humidity
Light intensity
CO2 concentration
Soil Moisture
Soil pH
Soil temperature
Image feed
The following sensors are connected to an Arduino Mega to measure and transmit the above sensor readings upon a command sent by the DE-10 Nano.
DHT11 - Environmental temperature and humidity
CJMCU-101 - Light intensity
MH-Z19C - CO2 concentration
Arducam 2MP Mini Plus - Image capture
EVAL-CN0398-ARDZ - Soil temperature, pH and moisture
A pH probe, a capacitive soil moisture sensor and an RTD sensor is connected to the EVAL-CN0398 Arduino shield by Analog Devices. The sensor measurements are read and converted to digital by the AD7124 ADC on the EVAL board. This communicates the sensor readings to the Arduino Mega through SPI interface.
Additionally the following actuators are connected to the Arduino Mega board to control the irrigation, ventilation and light requirements.
Water sprinklers powered by a submersible DC motor
12V DC Fan
Addressable and water-proof LED strips
We use Bluetooth technology to transfer data between the Arduino Mega and the controller DE-10 Nano. We designed our system to be less dependent on a continuous internet connection for it to be adoptable by domestic users and farmers even without a stable internet connection. Also, the power consumption is lower in Bluetooth than in Wi-Fi. [7]
An HC-05 bluetooth module is connected to the Arduino Mega to communicate with the DE-10 Nano. Upon specified commands sent from the DE-10 Nano over Bluetooth, the Arduino will read and send back the sensor data over Bluetooth and enable or disable the actuators.
Control System Design
As a further improvement to the previous implementation, we are working on modifying our design in the following way.
We replaced the standalone HC-05 with a RFS daughter card with a Bluetooth module. We referred to the RFS Bluetooth tutorial based on the Nios II softcore processor given in the user manual of the RFS. It integrates the RS232 UART IP block offered by Intel which is far superior and rigorously tested. We modified and integrated the UART with an on chip RAM and GPIO inputs to hard reset and change modes of the system. All the IP blocks, along with the Intel hard processor system (HPS) were integrated using the Platform Designer tool offered by Intel Quartus Prime. Later, it was synthesized and all the header files were generated to be used to write code to control the modules integrated in the FPGA portion through the HPS.
Following steps were followed in this implementation.
1. Creating the hardware design
Get the Golden Hardware Reference Design(GHRD)
Modifying the GHRD using Qsys tool (Platform Designer)
Adding components such as HPS, Bluetooth UART to the hardware
Generating HDL
Synthesizing the system and generating a bitstream
2. Generating a preloader
Configuring the preloader
Compiling the preloader
3. Setting up U-Boot
Setting up GCC toolchain
Compiling U-Boot
Setting up boot script
4. Generating and compiling the device tree
5. Testing the system
Creating an image file and mounting it
Creating partitions
Creating the file systems
Write the SD card image to a SD card
Testing
6. Configuring and compiling the Linux kernel
Setting up GCC 7.x toolchain
Writing the Linux kernel source code
Configuring the Linux kernel
Compiling the kernel
7. Generating the root file system
Configuring Buildroot
Configuring BusyBox
Generating the root file system
8. Generating a header file with the addresses of the HPS
9. Develop and deploy the source code
We have completed the hardware design. Next, we will move onto develop the source code in HPS using the above method.
The following diagram shows the Platform Designer system design.
The FPGA utilization after compilation is shown below.
The RTL view of the system is illustrated below.
Why do we use this design approach?
Easier to control and improve
Benefit of the GUI for the system designers and the end user
Driver and system functions are handled by Linux
As mentioned before, we chose Bluetooth technology due to its advantages over Wi-Fi for a system of green machines within a range of less than 10m. But as both Bluetooth and Wi-Fi technologies are embedded in the RFS daughter card, we can switch to Wi-Fi easily without much design changes, if the need arises to expand the coverage of the system.
HPS Implementation
The generated header files for the HPS will be used to write the source code for the control system using C++. We are currently working on modifying our code accordingly.
The image data will be fed into the neural network which is explained in detail in the next section. The classification data from the neural network and the sensor data will be processed by the control C++ program to determine appropriate requirements of water, light and ventilation and write the control signals for the actuators to the Arduino via Bluetooth.
The daily irrigation water requirement for the plants are calculated using the equation below.
IWR = As x (I - S)
IWR - Irrigation Water Requirement
As - Target Surface Area
I - Irrigation Applied
S - Difference in Moisture
[8]
If the soil moisture level falls below a threshold, the irrigation system will be turned on automatically for a predetermined duration.
Ventilation to the greenhouse is provided by turning on a fan that circulates air. This is determined by the temperature and humidity measurements and carbon dioxide level within the greenhouse. Minimum and maximum set points for temperature and humidity are determined according to the experiments and plant data. The mid-point is taken as the target value to be maintained within the greenhouse. The fan is turned on when the temperature or humidity falls below the minimum set point or carbon dioxide level is higher than recommended and turned on when they rise above the maximum set point or carbon dioxide level is lower than recommended. [8]
For chili plants;
Temperature - 26 - 30 Celcius
Humidity - 70% - 80%
CO2 - 1000 - 1500 ppm
The algorithm is illustrated in the Software Design Flow section.
Neural Network Accelerator
We implement a neural network on the DE-10 SoC to process the images obtained from the plants and detect abnormalities and degrading conditions.
Neural Network Structure
We use a convolutional neural network with 5 neural layers and 4 support layers. There are 3 three convolution layers accompanied by max-pool layers. The details are as follows,
• Conv1: 11x11 kernel, stride 4, 8 channels
• Max1: 2x2 max pooling
• Conv2: 9x9 kernel, stride 2, 16 channels
• Max2: 2x2 max pooling
• Conv3: 5x5 kernel, stride 2, 16 channels
• Max3: 2x2 max pooling
Next, a flatten layer converts the 2-dimensional data to 1-dimensional data to be processed by 2 fully connected layers. The first fully connected layer (F1) has 128 nodes with ReLu activation, and the output fully connected layer has 2 outputs with Sigmoid activation. The neural network decides whether the plant needs normal attention or special attention during wilting or disease. In future, this can be extended to separately identify wilting and each disease condition faced by different plant species.
Implementation
The above neural network is developed using OpenCL SDK for Intel FPGAs. The reason for selecting OpenCL was because of the ability to write a single program to execute the sequential process of the CNN on the ARM HPS and to perform parallel convolutional multiplication on the FPGA for acceleration.
As the first step, the following 4 kernels are developed using OpenCL C on OpenCL SDK and compiled using the AOC to generate the OpenCL executable file (.aocx) which can be downloaded to the FPGA.
11x11 Convolutional OpenCL kernel
9x9 Convolutional OpenCL kernel
5x5 Convolutional OpenCL kernel
2x2 Max pool OpenCL kernel
Next, the host program is written using C/C++ OpenCL and cross compiled to an executable application with Intel SoC-EDS.
The design flow of the implementation of the neural network is illustrated below.
Cloud Connectivity
We designed an Microsoft Azure IoT Hub based custom IoT solution to store and continuously process the data obtained from the sensors. This solution makes use of Azure as a platform as a service(PaaS). The DE-10 Nano board was provisioned to an Azure IoT Edge module which sends the telemetry sensor readings obtained by the FPGA. This IoT edge module will also be used to configure the FPGA remotely using container based applications to provide updates based on the results and observations of data obtained in the long term.
The following figure illustrates how the Microsft Azure based management system is designed.
The following images show the process of provisioning DE10 Nano as an IoT Edge device.
However, after this step, an error occured while connecting RFS board to obtain the telemetry of the sensor data. Therefore, in the current implementation, ethernet was used to transfer the data into the database.
The data obtained by the Azure IoT Hub will be stored in a Cosmos DB database. The following features of Cosmos DB were considered while selecting it as our database system.
Ability to ingest device telemetry at high data rates
Queries with low latency and high availability
The following figure shows sample sensor data stored in the implemented Cosmos DB database system.
Additionally, as we expand our system to be compatible with more variations of plants, the pre-trained weights for the neural network for each plant type will be stored in a separate database system on Cosmos DB. The weights of the neural network corresponding to the selected plant could be fetched from the Cosmos DB database using a python application run on the HPS and stored in the memory of the SoC.
6. Performance metrics, performance to expectation
Our mini greenhouse system has been designed to optimize the performance of the agricultural field in several aspects.
Power Consumption < 18W
This value was measured when all the actuators within a green machine were turned on at rated full power.
This can be quantitatively measured by comparing the ratio (yield/power consumption) of two similar systems implemented with and without FPGAs. As a future step in our project, we plan to conduct further analysis of the energy consumption and optimization using the Power Monitor LTC2945IDE provided by Linear Technology through Analog Devices.
Resource Savings
- No pesticides required
The plants are protected from pests within the controlled environment
- Only natural fertilizers were used
Only compost was used as a nutrient source. Synthetic fertilizers were not used at all
- Water saved by 20% - 40%
Water requirement was measured for manual watering twice a day and automatic watering using the system for the plants spread in the area within the green machine. 20% - 40% water saving was observed. Also, the humidity within the green machine is higher when the automatic sprinkler system is used which favors the chili plants.
- 80% of abnormalities detected
The neural network was implemented to detect abnormalities in plants like bent plants, discolored leaves and fruits. The neural network was trained using a set of 3500 images collected from the plants and a 80% accuracy was achieved in detecting such abnormalities.
CNN Performance
- Accuracy of training set = 81.04%
- Accuracy of test set = 80.45%
To analyze the performance of our system, we plan to conduct an evaluation by growing the same kind of plant in the optimal environment within a green machine and the normal outdoor environment and measure the growth and final yield.
7. Sustainability results, resource savings achieved
The system deisgn scheme, hardware block diagram and the software flow is explained in detail in the above section. The following digrams summarize them.
System Design Scheme
Hardware Block Diagram
Software Flow
Sustainability Results Achieved
As mentioned earlier, our project focuses on two SDG goals. The following sustainability results related to those SDGs could be achieved by the current implementation of our mini greenhouse.
Qualitative Results Achieved
-
Implementing a smart and efficient management of a mini greenhouse
Our system was designed to be always optimized for better resource management and to achieve maximum yield possible. This increased the productivity of agriculture and the resources could be managed intelligently.
Therefore, this paved a way for the urban community to contribute to agriculture. Our domestic users can use our mini greenhouse to maintain a garden within their homes to grow some portion of their food requirement. This reduces the demand from these households and, in turn, the agricultural products from small to large scale farms can be distributed among the less fortunate urban communities and rural communities that lack access to safe food.
As our system is fully automated, the labor needed to maintain such a cultivation is minimized. Therefore, the farmers and domestic users can save much of money spent for labor charges and resources.
The farmers who are young will make more investments in automation with much interest than the older farmers. The production of customized and rare plants will be encouraged within the newer generations, which will improve the diversity of products and increase the revenue.
-
Mental health
Our greenhouse system was designed to be attractive for exhibition purposes. As a result, maintaining a mini home garden within their own homes, improved the mental and physical well-being of the users. They can enjoy a fruitful garden and save time otherwise spent to manage a garden manually. Our green machine is presentable as a valuable achievement of the users to grow their own food and it encourages their family and friends to try it out themselves.
Quantitative Results Achieved
-
Prevent excessive use of chemicals - No pesticides required
By maintaining optimal conditions in the controlled environment, the plants are less prone to contact diseases. Therefore, the pesticides need not be used at all which reduces the risk of consuming harmful chemicals. This will allow the farmers to save expenses spent on pesticides and allow them to offer their food products for a lower price to be more affordable by consumers. Also, this will prevent the degradation of soil due to long term exposure to these chemicals and reduce its environmental impact. As a future step in our project, by measuring the nutrient level in the soil using an NPK sensor, we would be able to efficiently provide the required natural nutrients for the plants mixed with water.
-
Encourage and increase organic food consumption - 100% organic food achieved
The consumption of organic food is encouraged by our mini greenhouse system by making gardening approachable to the urban community. Buying organic food from markets is very expensive and unaffordable by most average domestic users. But we were able to design our mini greenhouse to be able to successfully grow plants avoiding toxic chemicals like pesticides. Also, since optimal natural conditions like light are provided to the plants to encourage growth, the requirement of chemical nutrients is minimized. This ensures food safety for the consumers and provides long term health benefits like increased lifetime and preventing cancer.
The research analysis conducted showed that smart agricultural applications reduce food wastage, water wastage, enhance soil management, reduce carbon discharge, reduce pesticide usage, improve food quality as well as revenue. Therefore, smart agriculture through systems like our green machine facilitate environmental,social and economic aspects of sustainability. [9]
-
Efficient use of water - 24% increased productivity with 20% less water
Our greenhouse system can determine the precise amounts of resources like water for the specific plant type that grows within it based on its type and stage of growth. The same efficiency can never be achieved manually because it is a very tiresome process and requires unnecessary labor. This, in turn, will increase the amount of freshwater available for human use which will lead to achieve SDG 12.
Our system was heavily influenced from a similar experiment done using microcontrollers. In that, they had observed a 20% reduction in the amount of water applied, 32% increase in the water user efficiency and 24% increase in productivity when using the irrigation control we used compared to conventional manual irrigation twice a day. [8]
-
Power Saving - <18W power consumption
We measured the power consumption to be 18W when all the actuators are turned on within a green machine. This value was measured without any optimization to the system. Due to the low power consumption of an FPGA compared to another controller performing the same computational task, the performance obtainable relative to the power consumed is maximized in a system like ours. We plan to reduce this power consumption further as a future development to the project.
Also, we plan to install a solar panel paired with a battery which can supply sufficient power to make the green machine completely self sustainable. This will let us produce food greatly at no cost at all. This makes the harmful emissions to the environment be minimal, ensuring the SDG 12, responsible consumption.
The other expected sustainability results in section 3 can also be achieved by implementing our mini greenhouse system on a larger scale.
8. Conclusion
The field of agriculture has the potential to increase its net produce by incorporating the latest technology available in the world. But not enough solutions have been introduced to the world that harness the power of such technologies to improve the quality and efficiency of agricultural processes.
The concept of green machines open up a new pathway for the urban households to engage in agriculture willingly and effortlessly. Also, it provides the farmers with an efficient way of managing their products by combining several of such green machine modules together. Also, by integrating the internet of things to the system, we are able to provide the data obtained from such green machines for research and other experiments that improve the quality of the agricultural yield.
When this concept is implemented on a larger scale, it is possible to create a local community within a vicinity with localized data from several green machines that grow different types of plants. The community can efficiently share their organic products with the other members of the community that improves their overall quality of life and food safety.
As future steps for our project, we plan to develop a website/mobile app for the users to monitor the health of their plants remotely, get recommendations about maintaining plants based on past and present data, and predictions about the yield. This web application will be based on Microsoft Azure Webapps.
Furthermore, as a next step in our project, we plan to improve our neural network to detect diseases and other defects.
Overall, this project has been a success with the help and resources provided by Terasic, Analog Devices and Microsoft Azure communities, for which we are very thankful.
References
[1] Tzounis, A., Katsoulas, N., Bartzanas, T. and Kittas, C., 2017. Internet of Things in agriculture, recent advances and future challenges. Biosystems Engineering, 164, pp.31-48.
[2] UNDP. 2022. Sustainable Development Goals | United Nations Development Programme. [online] Available at: <https://www.undp.org/sustainable-development-goals?utm_source=EN&utm_medium=GSR&utm_content=US_UNDP_PaidSearch_Brand_English&utm_campaign=CENTRAL&c_src=CENTRAL&c_src2=GSR&gclid=Cj0KCQjwmPSSBhCNARIsAH3cYgZ14ieHOp6IAA873OgaD2KfGJxhqWptLzAksDcPtJwDeJu5AaEcImUaArS7EALw_wcB#zero-hunger> [Accessed 19 April 2022].
[3] Fuglie, Keith, Madhur Gautam, Aparajita Goyal, and William F. Maloney. 2020. Harvesting Prosperity: Technology and Productivity Growth in Agriculture. Washington, DC: World Bank. doi:10.1596/978-1-4648-1393-1. License: Creative Commons Attribution CC BY 3.0 IGO
[4] Jha, K., Doshi, A., Patel, P. and Shah, M., 2019. A comprehensive review on automation in agriculture using artificial intelligence. Artificial Intelligence in Agriculture, 2, pp.1-12.
[5] Khan, W., Ahmed, E., Hakak, S., Yaqoob, I. and Ahmed, A., 2019. Edge computing: A survey. Future Generation Computer Systems, 97, pp.219-235.
[6] Thirer, N. and Uchansky, I., 2015. An FPGA Based Computer System for Greenhouse Control. ATHENS JOURNAL OF SCIENCES, 2(1), pp.23-32.
[7] Navarro, E., Costa, N. and Pereira, A., 2020. A Systematic Review of IoT Solutions for Smart Farming. Sensors, 20(15), p.4231.
[8] Sagheer, A., Mohammed, M., Riad, K. and Alhajhoj, M., 2020. A Cloud-Based IoT Platform for Precision Control of Soilless Greenhouse Cultivation. Sensors, 21(1), p.223.
[9] Maroli, A., Narwane, V. and Gardas, B., 2021. Applications of IoT for achieving sustainability in the agricultural sector: A comprehensive review. Journal of Environmental Management, 298, p.113488.
[10] Kalyani, Y. and Collier, R., 2021. A Systematic Survey on the Role of Cloud, Fog, and Edge Computing Combination in Smart Agriculture. Sensors, 21(17), p.5922.
[11] Jha, K., Doshi, A., Patel, P. and Shah, M., 2019. A comprehensive review on automation in agriculture using artificial intelligence. Artificial Intelligence in Agriculture, 2, pp.1-12.
[12] Chowdhury, M., Khandakar, A., Ahmed, S., Al-Khuzaei, F., Hamdalla, J., Haque, F., Reaz, M., Al Shafei, A. and Al-Emadi, N., 2022. Design, Construction and Testing of IoT Based Automated Indoor Vertical Hydroponics Farming Test-Bed in Qatar.
[13] Shirsath, P., Kamble, P., Mane, R., Kolap, A. and More, P., 2017. IOT Based Smart Greenhouse Automation Using Arduino. International Journal of Innovative Research in Computer Science & Technology, 5(2), pp.234-238.
18 Comments
Please login to post a comment.
Chirantha Jayampath Kurukulasuriya
Brilliant and inspiring work! Best of luck!
Ramith Hettiarachchi
Amazing Project! All the best!
Imalsha Liyanage
Outstanding job!
Best of luck!
Nethmi Buddhadasa
Great work!
Dilani Widanapathiranage
Great work! Good luck!!!
Risini Ranaweera
Hats off to the creativity! Good luck
Hiruni Wijewardena
A great initiative
Good luck!
Senuri
Great work!
Venodi Widanagamage
Brilliant innovation to sustain the agriculture! Keep up the good work!